Neural Network Topology Optimization Method Based on Three-way Decision
A neural network and optimization method technology, applied in the field of neural network topology optimization, can solve problems such as increasing model complexity and reducing model efficiency, and achieve the effect of solving unbalanced classification difficulty, improving accuracy, and compact network structure
- Summary
- Abstract
- Description
- Claims
- Application Information
AI Technical Summary
Problems solved by technology
Method used
Image
Examples
Embodiment 1
[0130] This embodiment is based on a three-branch decision-making neural network structure optimization method, which is used in the classification of Online NewsPopularity data. The specific process is:
[0131] Step 1: Initialize parameters
[0132] According to the ratio of 8:1:1, the Online News Popularity data set with 39,797 entries in the binary classification is divided into a training set with a size of (31837,61), a validation set with a size of (3980,61), and a validation set with a size of (3980 , 61) test set; select the activation function as the Swish function and the situation where the initialization parameters obey the normal distribution, and initialize the weights and biases of the neural network.
[0133]Step 2: In this embodiment, the neural network structure is SFNN, a hidden layer node is set, and the learning process of the neural network is realized on the training set
[0134] Step 2-1: The forward learning process of the neural network
[0135] In...
Embodiment 2
[0158] In this embodiment, the neural network structure optimization method based on the three-branch decision is applied to classification-related research fields such as medical image classification and spam filtering, so as to improve classification accuracy.
[0159] What is not mentioned in the present invention is applicable to the prior art.
PUM
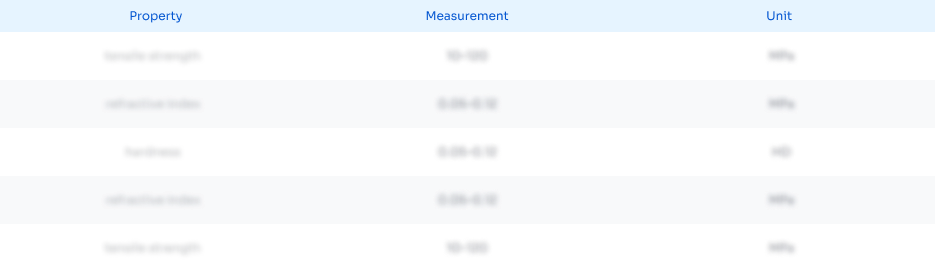
Abstract
Description
Claims
Application Information
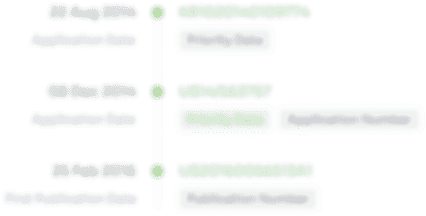
- R&D Engineer
- R&D Manager
- IP Professional
- Industry Leading Data Capabilities
- Powerful AI technology
- Patent DNA Extraction
Browse by: Latest US Patents, China's latest patents, Technical Efficacy Thesaurus, Application Domain, Technology Topic, Popular Technical Reports.
© 2024 PatSnap. All rights reserved.Legal|Privacy policy|Modern Slavery Act Transparency Statement|Sitemap|About US| Contact US: help@patsnap.com