Vehicle operation parameter prediction method and system containing space-time characteristics, electronic equipment and readable storage medium
A technology of vehicle operation and spatiotemporal characteristics, applied in the field of intelligent transportation, can solve the problems of lack of semantic information in the area, destroy the integrity of urban areas, and difficult to capture spatial correlation, and achieve the effect of improving the prediction results.
- Summary
- Abstract
- Description
- Claims
- Application Information
AI Technical Summary
Problems solved by technology
Method used
Image
Examples
Embodiment 1
[0060] Urban private car travel flows consist of the total number of vehicles entering, staying and leaving urban areas. Its travel is affected by regional functions and the dynamic interaction of travel flows between regions. For example, the travel flow of office areas during the day is usually more than that of residential areas; There is a lot of traffic. The dynamic interaction of private car travel flow between regions also reflects the travel rules of private cars and the relationship between regions. How to extract the spatio-temporal characteristics of private car travel is the first challenge. Furthermore, in this embodiment, the travel flow forecast for private cars includes the following steps:
[0061] Step 1: Construct a multi-view space-time map of the study area
[0062] Among them, the dynamic association between private car travel and urban areas is modeled as a multi-view space-time graph, which specifically includes distance graph, similarity graph, func...
Embodiment 2
[0106] Considering that the residence time of private cars reflects the attractiveness of the area to users, for example, the longer the user stays, the more attractive the area is to the user. How to make full use of the dwell time characteristics of private car users is the second challenge. For this reason, on the basis of Example 1, the dwell time is used to weight the spatio-temporal features, for any vertex v in the spatio-temporal graph i , the output sequence H of the multi-graph convolutional gate recurrent network 1 [i],...,H t [i]...,H T [i], T is the total number of time periods, the formula is as follows:
[0107]
[0108]
[0109]
[0110] Among them, W i,st Indicates the average residence time of vehicles in the i-th AOI area in the previous τ research period, τ is the number of research periods before the current research period t, u is the weight vector, e i is the attention vector of vertex i, a i is the weight vector of the attention network c...
Embodiment 3
[0113] In real life, other external factors such as weather, holidays and events will also affect the distribution of private car travel flow, and these factors make the prediction problem more difficult. Therefore, on the basis of Embodiment 1 or Embodiment 2, this Embodiment 3 also considers adding external features corresponding to external factors, and merging external features with spatiotemporal features as the input of joint prediction, and then transforming to obtain prediction results, that is, research The vehicle operating parameters of each AOI area in the time period.
[0114] In this embodiment, the conversion formula of the following fully connected network is selected:
[0115]
[0116] Among them, E t is the external feature, W is the learned weight, σ is the activation function, such as the Sigmoid function, and b is the bias item.
[0117] It should be understood that before the actual application, the model needs to be trained. After the present invent...
PUM
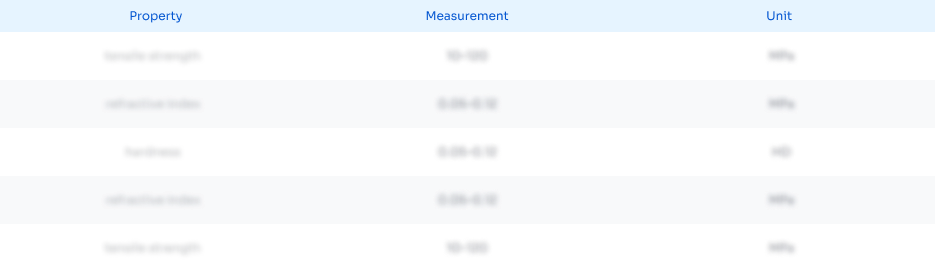
Abstract
Description
Claims
Application Information
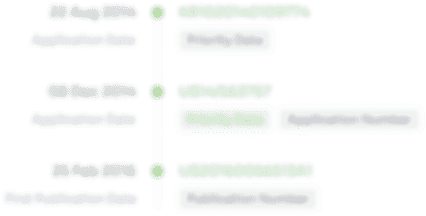
- R&D Engineer
- R&D Manager
- IP Professional
- Industry Leading Data Capabilities
- Powerful AI technology
- Patent DNA Extraction
Browse by: Latest US Patents, China's latest patents, Technical Efficacy Thesaurus, Application Domain, Technology Topic, Popular Technical Reports.
© 2024 PatSnap. All rights reserved.Legal|Privacy policy|Modern Slavery Act Transparency Statement|Sitemap|About US| Contact US: help@patsnap.com