An Attack Judgment Method for Fooling Interpretable Algorithms of Deep Neural Networks
A technology of deep neural network and explaining algorithm, which is applied in the field of attack judgment to fool the explainable algorithm of deep neural network, and achieves good robustness
- Summary
- Abstract
- Description
- Claims
- Application Information
AI Technical Summary
Problems solved by technology
Method used
Image
Examples
Embodiment Construction
[0059] The present invention will be further described below with reference to the accompanying drawings and embodiments.
[0060] An example of the complete method implementation according to the content of the present invention and its implementation are as follows:
[0061] The present invention is implemented on the deep neural network VGG19 model trained on the ImageNet data set. Taking Grad-CAM as an example, the detailed description is as follows:
[0062] 1) Generate a random initialization noise and generate a binary mask, if it is a single image of a single target object, such as figure 2 As shown in the first column, set the value of the corresponding square area to 0, and other areas to 1; if it is an image of a single multi-target object, such as Figure 5 As shown in the first column, the value corresponding to the position of the two square areas is set to 0 at the same time, and the other areas are set to 1.
[0063] 2) Multiply the noise and the binary mask...
PUM
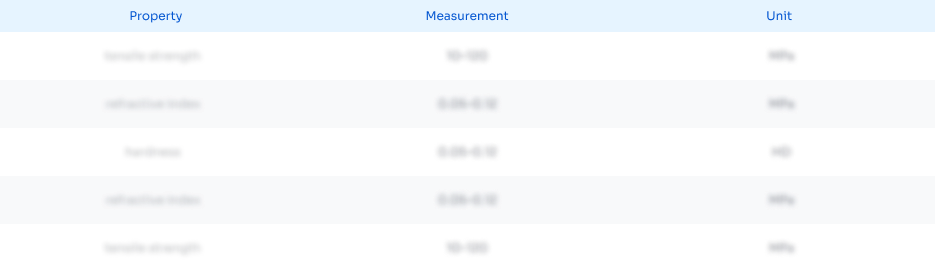
Abstract
Description
Claims
Application Information
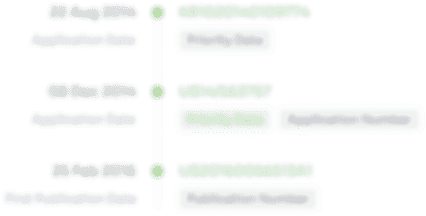
- R&D Engineer
- R&D Manager
- IP Professional
- Industry Leading Data Capabilities
- Powerful AI technology
- Patent DNA Extraction
Browse by: Latest US Patents, China's latest patents, Technical Efficacy Thesaurus, Application Domain, Technology Topic, Popular Technical Reports.
© 2024 PatSnap. All rights reserved.Legal|Privacy policy|Modern Slavery Act Transparency Statement|Sitemap|About US| Contact US: help@patsnap.com