Semi-supervised image classification method based on generative adversarial network
A classification method and semi-supervised technology, applied in biological neural network models, neural learning methods, instruments, etc., can solve problems such as limited application range and single image features, and achieve the goals of reducing dependencies, improving classification accuracy, and good classification accuracy Effect
- Summary
- Abstract
- Description
- Claims
- Application Information
AI Technical Summary
Problems solved by technology
Method used
Image
Examples
Embodiment Construction
[0026] The present invention will be further described in detail below in conjunction with the accompanying drawings and specific embodiments.
[0027] refer to figure 1 , the present invention comprises the following steps:
[0028] Step 1) Obtain training sample set and test sample set:
[0029] Step 1a) The number of categories obtained is K and each category contains A data set of images, and normalize the data set to obtain a normalized data set containing S normalized images, where 2≤K≤20, S≥60000; in this example, the number of categories is 10 And each category contains a cifar10 data set of 6000 images, normalize the data set, and obtain a normalized data set containing 60000 normalized images;
[0030] Step 1b) Randomly select n normalized images from each category of the normalized data set, and use the selected N normalized images as a test sample set, and then select l in the remaining S-N normalized images Label the frames to get the labeled training sample ...
PUM
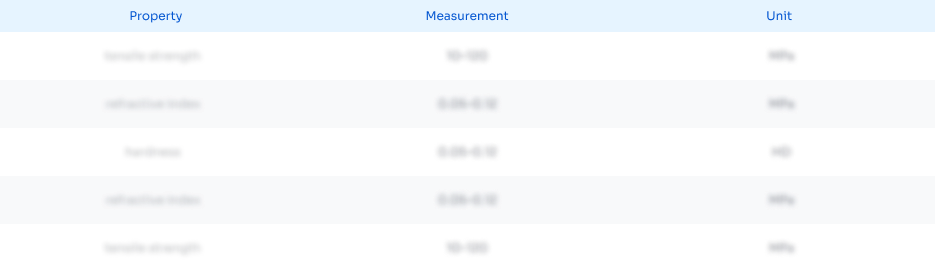
Abstract
Description
Claims
Application Information
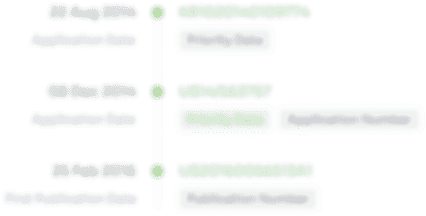
- R&D
- Intellectual Property
- Life Sciences
- Materials
- Tech Scout
- Unparalleled Data Quality
- Higher Quality Content
- 60% Fewer Hallucinations
Browse by: Latest US Patents, China's latest patents, Technical Efficacy Thesaurus, Application Domain, Technology Topic, Popular Technical Reports.
© 2025 PatSnap. All rights reserved.Legal|Privacy policy|Modern Slavery Act Transparency Statement|Sitemap|About US| Contact US: help@patsnap.com