Small sample industrial product defect classification method based on two-stage transfer learning
A technology for classification of industrial products and defects, applied in the field of visual inspection, can solve the problems of small number of defect samples, poor adaptability, and difficulty in collection, so as to improve the classification accuracy, improve the representation ability, and improve the efficiency of use.
- Summary
- Abstract
- Description
- Claims
- Application Information
AI Technical Summary
Problems solved by technology
Method used
Image
Examples
Embodiment Construction
[0023] In order to make the object, technical solution and advantages of the present invention clearer, the present invention will be further described in detail below in conjunction with the accompanying drawings and embodiments. It should be understood that the specific embodiments described here are only used to explain the present invention, not to limit the present invention. In addition, the technical features involved in the various embodiments of the present invention described below can be combined with each other as long as they do not constitute a conflict with each other.
[0024] combine figure 1 As shown, the two-stage migration learning model training strategy of the present invention is as follows: first, the negative samples in the original data set with positive and negative sample balance caused by fewer negative samples are expanded by 2-3 times through image data enhancement, and then the large number Randomly select a number of positive samples equivalen...
PUM
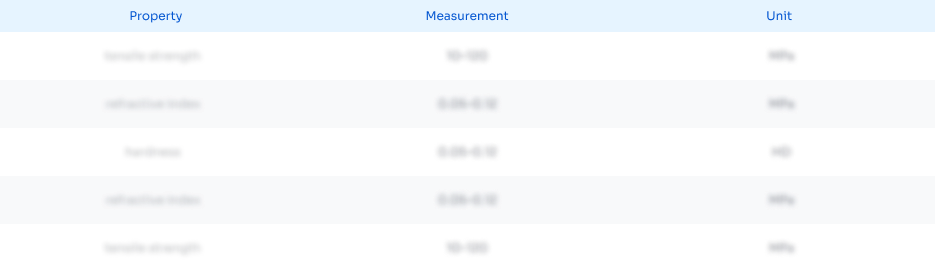
Abstract
Description
Claims
Application Information
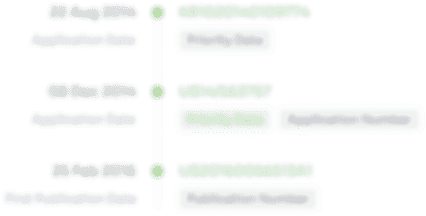
- R&D Engineer
- R&D Manager
- IP Professional
- Industry Leading Data Capabilities
- Powerful AI technology
- Patent DNA Extraction
Browse by: Latest US Patents, China's latest patents, Technical Efficacy Thesaurus, Application Domain, Technology Topic, Popular Technical Reports.
© 2024 PatSnap. All rights reserved.Legal|Privacy policy|Modern Slavery Act Transparency Statement|Sitemap|About US| Contact US: help@patsnap.com