A Self-Supervised Learning Fusion Method for Multiband Images
A fusion method and supervised learning technology, applied in the field of image fusion, can solve problems such as lack of labeled images and limited fusion results
- Summary
- Abstract
- Description
- Claims
- Application Information
AI Technical Summary
Problems solved by technology
Method used
Image
Examples
Embodiment Construction
[0027] The self-supervised learning fusion method of multi-band images based on multi-discriminators includes the following steps:
[0028] The first step is to design and build a generative adversarial network: design and build a multi-discriminator generative adversarial network structure. The multi-discriminator generative adversarial network consists of a generator and multiple discriminators; taking n-band image fusion as an example, a generator and n discriminators.
[0029] The generator network structure consists of a feature enhancement module and a feature fusion module. The feature enhancement module is used to extract the features of the source images of different bands and enhance them to obtain multi-channel feature maps of each band. The feature fusion module uses the merge connection layer in the channel. Feature connection is performed in dimension and the connected feature map is reconstructed into a fusion image, as follows:
[0030] The feature enhancement...
PUM
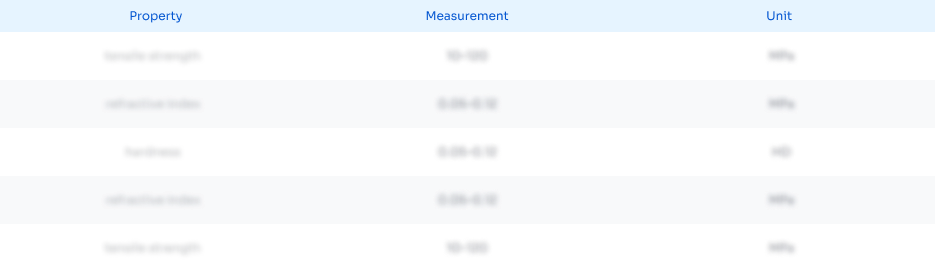
Abstract
Description
Claims
Application Information
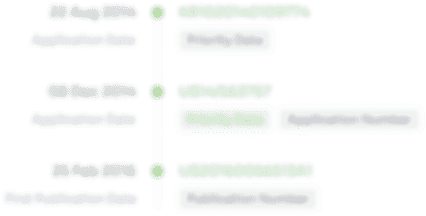
- R&D Engineer
- R&D Manager
- IP Professional
- Industry Leading Data Capabilities
- Powerful AI technology
- Patent DNA Extraction
Browse by: Latest US Patents, China's latest patents, Technical Efficacy Thesaurus, Application Domain, Technology Topic.
© 2024 PatSnap. All rights reserved.Legal|Privacy policy|Modern Slavery Act Transparency Statement|Sitemap