Discretized differentiable neural network search method based on entropy loss function
A search method and neural network technology, applied in neural learning methods, biological neural network models, neural architectures, etc., can solve the problems of small number of pruned edges, inaccuracy, and inability to guarantee discarded weights, etc., to reduce the loss of discretization accuracy , significant effect
- Summary
- Abstract
- Description
- Claims
- Application Information
AI Technical Summary
Problems solved by technology
Method used
Image
Examples
Embodiment approach
[0029] According to a preferred embodiment of the present invention, the constructed search space is a unit-based hypernetwork search space, denoted as O, where each element is a fixed operation, denoted as o(*).
[0030] In a further preferred embodiment, the supernetwork is composed of 8 cell structures stacked, including 6 normal cells and 2 reduction cells;
[0031] The initial channel number of each unit is 16, including 6 nodes, and the alternative operations for node connection include 7.
[0032] Preferably, the operation is 3x3 and 5x5 atrous separable convolution, 3x3 and 5x5 separable convolution, 3x3 average pooling, 3x3 maximum pooling and cross-layer connection.
[0033] Among them, within each unit, the purpose of the search is to determine an operation for each pair of nodes.
[0034] In the present invention, as figure 1 As shown, record (i, j) as a pair of nodes, where 0≤i≤j≤N-1, N is the number of input edges reserved for each node;
[0035] According to ...
Embodiment 1
[0134] 1. Database:
[0135] The commonly used CIFAR10 and ImageNet datasets are used to evaluate the network architecture search method described in the present invention. Among them, CIFAR10 consists of 60,000 images with a spatial resolution of 32×32. The images are evenly distributed across 10 categories, with 50,000 training images and 100,000 test images; ImageNet contains 1,000 categories, including 1.3 million high-resolution training images and 50,000 validation images. These images are uniformly distributed across the class.
[0136] According to the commonly used settings, the mobile setting is adopted. In the test phase, the input image size is fixed at 224×224, and the structure is searched on CIFAR10 and then migrated to the ImageNet dataset.
[0137] 2. Compare the classification errors of the network structures searched by various search methods in the present invention and the prior art on the CIFAR10 data set, and the results are as shown in Table 1:
[01...
experiment example 1
[0155] Under different target network configurations, the DARTS of prior art and the search method described in the present invention (DA 2 S) search results are compared, and the results are shown in Table 4.
[0156] Table 4
[0157]
[0158] It can be seen from Table 4 that under different configurations, DARTS has a great loss of precision in the discretization process, while the search method of the present invention has a great improvement in the loss of precision, decreasing from [77.75-78.00] to [0.21-21.29].
[0159] further, Figure 10 Shows the change curve of the softmax value of the operation weight of the method DARTS in the standard unit on CIFAR10 during the search process; Figure 11 Shows the change curve of the softmax value of the operation weight in the descending unit of the method DARTS on CIFAR10 during the search process; Figure 12 It shows the network structure searched when the method DARTS is configured on CIFAR10 to select 3 out of 14 items...
PUM
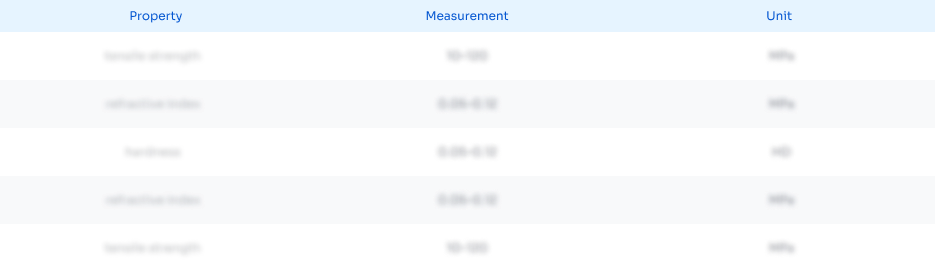
Abstract
Description
Claims
Application Information
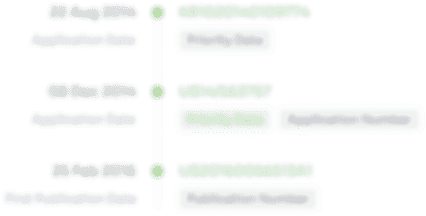
- R&D Engineer
- R&D Manager
- IP Professional
- Industry Leading Data Capabilities
- Powerful AI technology
- Patent DNA Extraction
Browse by: Latest US Patents, China's latest patents, Technical Efficacy Thesaurus, Application Domain, Technology Topic, Popular Technical Reports.
© 2024 PatSnap. All rights reserved.Legal|Privacy policy|Modern Slavery Act Transparency Statement|Sitemap|About US| Contact US: help@patsnap.com