Monitoring data exception diagnosis method based on generative adversarial network
A technology for monitoring data and anomaly diagnosis, applied in biological neural network models, neural learning methods, instruments, etc., can solve problems such as massive and complex information processing capabilities that need to be improved, and achieve automatic classification, simplified learning methods, and small overlap Effect
- Summary
- Abstract
- Description
- Claims
- Application Information
AI Technical Summary
Problems solved by technology
Method used
Image
Examples
Embodiment 1
[0035] Embodiment 1: see Figure 1-Figure 3 , a monitoring data anomaly diagnosis method based on a generative confrontation network, the method comprising the following steps: Step 1: training data preparation stage, step 2: network training stage, and step 3: data anomaly diagnosis stage.
[0036] Step 1: training data preparation stage, this stage includes two steps:
[0037] Step 1-1: Determine the minimum sampling frequency of the required data according to the analysis purpose, perform resampling operation on the data recorded by the structural health monitoring system (SHMS) to be detected, and down-sample the original data without losing the original data. inherent structural information;
[0038] Step 1-2: Set the basic time interval, divide the measured data into several sub-segments, perform normalization operation on the data of each sub-segment according to formula (3), and normalize it to the interval [-1,1]. Then, the dataset of each subsegment is converted in...
PUM
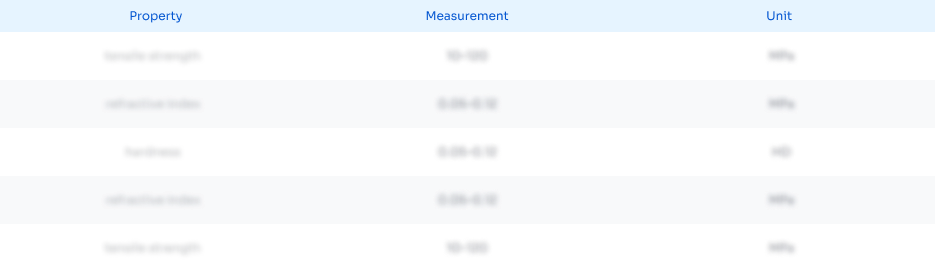
Abstract
Description
Claims
Application Information
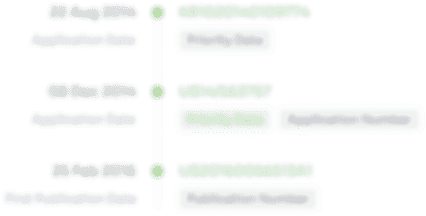
- Generate Ideas
- Intellectual Property
- Life Sciences
- Materials
- Tech Scout
- Unparalleled Data Quality
- Higher Quality Content
- 60% Fewer Hallucinations
Browse by: Latest US Patents, China's latest patents, Technical Efficacy Thesaurus, Application Domain, Technology Topic, Popular Technical Reports.
© 2025 PatSnap. All rights reserved.Legal|Privacy policy|Modern Slavery Act Transparency Statement|Sitemap|About US| Contact US: help@patsnap.com