Multi-model target state prediction method and system based on deep reinforcement learning
A technology of reinforcement learning and target state, applied in radio wave measurement system, calculation model, machine learning, etc., can solve problems such as large prediction error, inability to cope with prediction accuracy, and short predictable time, so as to improve the length of time and improve the goal Effect of State Prediction Accuracy
- Summary
- Abstract
- Description
- Claims
- Application Information
AI Technical Summary
Problems solved by technology
Method used
Image
Examples
Embodiment Construction
[0025] The present invention will be described in further detail below in conjunction with the embodiments given in the accompanying drawings.
[0026] Such as figure 1 As shown, the environmental target state scene environment involved in the present invention includes target T, historical states St (t=0, 1, 2...N) of each target, road physical boundary information, and road line marking information. The target T mainly includes: historical position information Pt of the target (t=0, 1, 2...N), historical shape information Lt of the target (t=0, 1, 2...N), historical orientation information Ot of the target (t= 0,1,2...N), historical speed information Vt of the target (t=0,1,2...N). Road physical boundary information Bt indicates impassable road boundaries, such as road railings, curbs, cliffs, etc. The road line marking information Mt represents the artificially drawn marking lines in the traffic rules, such as real lane lines, virtual lane lines, zebra crossings, stop lin...
PUM
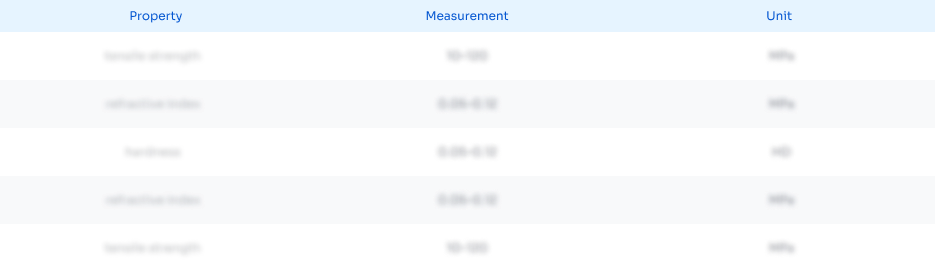
Abstract
Description
Claims
Application Information
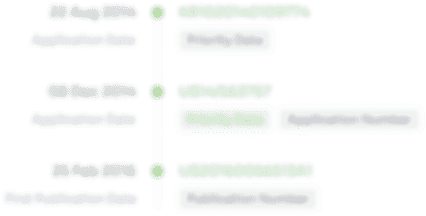
- R&D Engineer
- R&D Manager
- IP Professional
- Industry Leading Data Capabilities
- Powerful AI technology
- Patent DNA Extraction
Browse by: Latest US Patents, China's latest patents, Technical Efficacy Thesaurus, Application Domain, Technology Topic, Popular Technical Reports.
© 2024 PatSnap. All rights reserved.Legal|Privacy policy|Modern Slavery Act Transparency Statement|Sitemap|About US| Contact US: help@patsnap.com