Unsupervised domain adaptive method combining deep attention features and conditional adversarial
A technology of attention and conditions, applied in computer parts, character and pattern recognition, instruments, etc., can solve problems such as negative transfer, achieve the goal of avoiding negative transfer, improving generalization ability and classification accuracy, and improving transferability Effect
- Summary
- Abstract
- Description
- Claims
- Application Information
AI Technical Summary
Problems solved by technology
Method used
Image
Examples
Embodiment Construction
[0040] The content of the invention will be further described below in conjunction with the accompanying drawings and the unsupervised domain adaptation on the dataset Office-31.
[0041] figure 1 It is a flow chart of an unsupervised domain domain adaptation method for joint depth attention features and conditional confrontation according to an embodiment of the present invention, including the following steps:
[0042] 1. Divide the image dataset to be processed into source domain and target domain.
[0043] 2. Design a transferable attention and conditional confrontation network for the image data set to be processed. We use the ResNet-50 basic network to extract the features of the image, and then transfer these features to the transferable attention network to obtain weighted features. Figure; Finally, these weighted feature maps are sent to the conditional confrontation network for training, and through continuous iterative training, an image classification model that c...
PUM
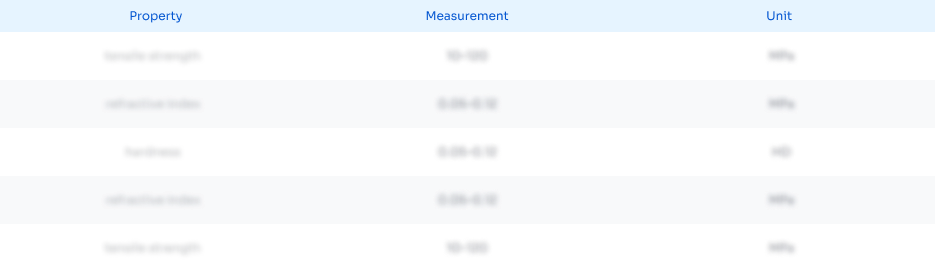
Abstract
Description
Claims
Application Information
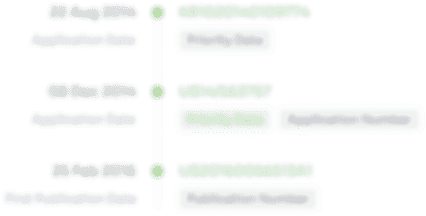
- R&D Engineer
- R&D Manager
- IP Professional
- Industry Leading Data Capabilities
- Powerful AI technology
- Patent DNA Extraction
Browse by: Latest US Patents, China's latest patents, Technical Efficacy Thesaurus, Application Domain, Technology Topic, Popular Technical Reports.
© 2024 PatSnap. All rights reserved.Legal|Privacy policy|Modern Slavery Act Transparency Statement|Sitemap|About US| Contact US: help@patsnap.com