Cooperative Estimation Method of Remaining Power of Li-ion Battery and Sensor Bias Based on Neural Network and Unscented Kalman Filter
A lithium electronic battery, traceless Kalman technology, applied in the measurement of electrical variables, instruments, measuring electricity, etc., can solve the problems of inability to online estimation, unsuitable for lithium electronic batteries, requiring several to ten hours, etc. The effect of small error, high precision and fast convergence speed
- Summary
- Abstract
- Description
- Claims
- Application Information
AI Technical Summary
Problems solved by technology
Method used
Image
Examples
Embodiment Construction
[0057] A method for cooperatively estimating the residual power of a lithium electronic battery and sensor bias based on a neural network and an unscented Kalman filter, comprising the following steps:
[0058] S1: Conduct lithium-ion battery charging and discharging experiments and collect sample data, including training data and test data;
[0059] S2: Determine the input and output variables of the neural network, and establish the RBFNN model of the SOC;
[0060] S3: Perform parameter learning on the established RBFNN based on the training data set to obtain an accurate RBFNN model;
[0061] S4: Use the test data to perform an independent accuracy test on the established RBFNN;
[0062] S5: Set the SOC as an internal state, and design RBFNN-UKF to realize real-time estimation of SOC when the initial SOC is uncertain;
[0063] S6: Set the sensor bias to the expanded state, and design the expanded RBFNN-UKF on the basis of the original RBFNN-UKF to realize the collaborativ...
PUM
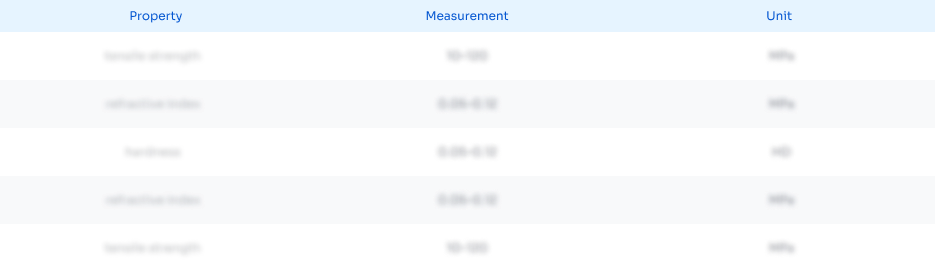
Abstract
Description
Claims
Application Information
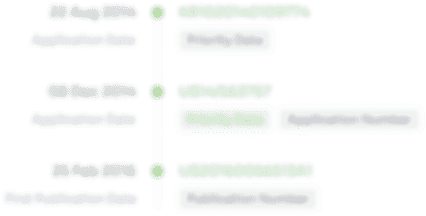
- R&D Engineer
- R&D Manager
- IP Professional
- Industry Leading Data Capabilities
- Powerful AI technology
- Patent DNA Extraction
Browse by: Latest US Patents, China's latest patents, Technical Efficacy Thesaurus, Application Domain, Technology Topic.
© 2024 PatSnap. All rights reserved.Legal|Privacy policy|Modern Slavery Act Transparency Statement|Sitemap