Rolling bearing fault diagnosis method based on multivariate time-shifting multi-scale permutation entropy
A technology for fault diagnosis and rolling bearings, which is applied in the testing of mechanical components, testing of machine/structural components, and measuring devices, etc. The effect of time-consuming calculation and high degree of fault identification
- Summary
- Abstract
- Description
- Claims
- Application Information
AI Technical Summary
Problems solved by technology
Method used
Image
Examples
Embodiment Construction
[0079] The inventive method comprises the steps:
[0080] (1) Collect the original fault vibration signal of the object to be diagnosed;
[0081] (2) Extract the multivariate time-shifted multi-scale permutation entropy of the original fault vibration signal;
[0082] (3) Using the Laplacian score method to reduce the dimensionality of the multivariate time-shifted multiscale permutation entropy, and obtain the fault feature samples after dimensionality reduction;
[0083] (4) divide the fault feature samples after dimensionality reduction into multiple training samples and test samples;
[0084] (5) adopt multiple training samples to train the multi-fault feature classifier based on the bat algorithm optimized support vector machine);
[0085] (6) Classify the test samples using the multi-fault feature classifier that has been trained;
[0086] (7) Identify the working state and fault type and degree of the object according to the classification result.
[0087] The inven...
PUM
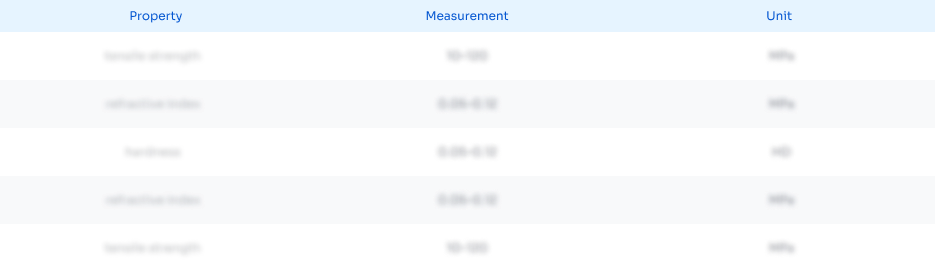
Abstract
Description
Claims
Application Information
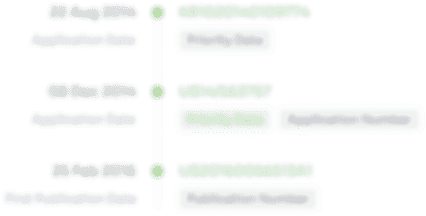
- R&D Engineer
- R&D Manager
- IP Professional
- Industry Leading Data Capabilities
- Powerful AI technology
- Patent DNA Extraction
Browse by: Latest US Patents, China's latest patents, Technical Efficacy Thesaurus, Application Domain, Technology Topic, Popular Technical Reports.
© 2024 PatSnap. All rights reserved.Legal|Privacy policy|Modern Slavery Act Transparency Statement|Sitemap|About US| Contact US: help@patsnap.com