Multi-behavior migration recommendation method based on deep learning
A recommendation method and deep learning technology, applied in the field of data mining and recommendation, can solve the problems of inability to dig deep into the deep features of users and items, lack of explicit feedback information, poor recommendation effect, etc., to achieve strong generalization ability and solve data problems Sparsity problem, the effect of solving data sparsity problem
- Summary
- Abstract
- Description
- Claims
- Application Information
AI Technical Summary
Problems solved by technology
Method used
Image
Examples
Embodiment Construction
[0032] The present invention will be described in further detail below in conjunction with the accompanying drawings. Based on various implicit behavior feedback information of users and items in the recommendation system, the present invention mines rich source behavior information and migrates it to relatively sparse target behavior, models user preferences, and solves the problem of data sparsity. Such as figure 1 Shown, the present invention specifically comprises the following steps:
[0033] Step 1: Obtain various implicit feedback data sets of users and process them.
[0034] (1) Obtain target user and item data from e-commerce websites, where the methods of obtaining data include web crawlers or cooperation methods. The obtained data is cleaned to extract implicit feedback data, wherein the implicit feedback data set mainly includes click data, data added to shopping cart, favorite data and purchase data. According to the user behavior data set, the triplet is forme...
PUM
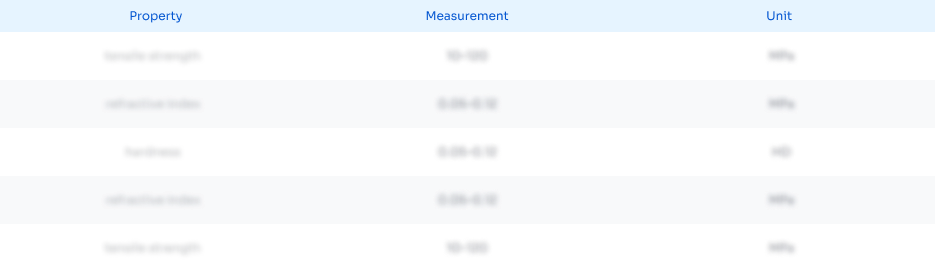
Abstract
Description
Claims
Application Information
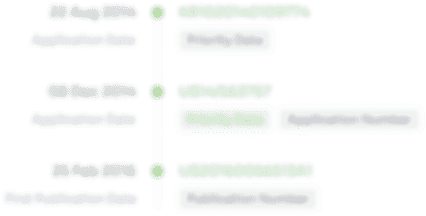
- R&D Engineer
- R&D Manager
- IP Professional
- Industry Leading Data Capabilities
- Powerful AI technology
- Patent DNA Extraction
Browse by: Latest US Patents, China's latest patents, Technical Efficacy Thesaurus, Application Domain, Technology Topic, Popular Technical Reports.
© 2024 PatSnap. All rights reserved.Legal|Privacy policy|Modern Slavery Act Transparency Statement|Sitemap|About US| Contact US: help@patsnap.com