DBN-GA model-based thermal power prediction method for solar heat collection system
A DBN-GA and solar heat collection technology, which is applied in genetic models, predictions, biological neural network models, etc., can solve problems such as the inability to explain the reasoning process and reasoning basis, the neural network cannot work normally, and the model cannot reflect the sample rules. Achieve the effect of optimizing resource utilization, maximizing benefits, and reducing training time
- Summary
- Abstract
- Description
- Claims
- Application Information
AI Technical Summary
Problems solved by technology
Method used
Image
Examples
Embodiment Construction
[0017] The present invention will be described in detail below in conjunction with the accompanying drawings and specific embodiments.
[0018] The thermal power prediction method of the present invention is based on the DBN-GA model, wherein the deep belief neural network (DBN) is a generative model, by training the weights between its neurons, the entire neural network is allowed to generate training data according to the maximum probability. It can not only identify features, classify data, but also use it to generate data, but because its learning process is too simple, there may be incomplete training defects in the training process; therefore, using genetic algorithm (GA) to optimize deep belief neural network The network solves this problem by combining error backpropagation and updating its weights until within the set error range, improving the accuracy of prediction, and is well applied in the thermal power prediction of solar thermal collection systems.
[0019] ref...
PUM
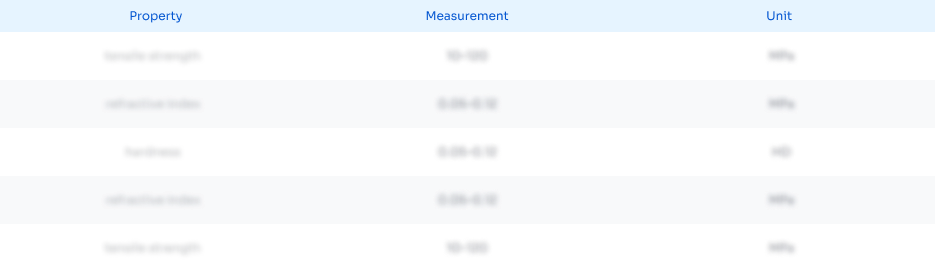
Abstract
Description
Claims
Application Information
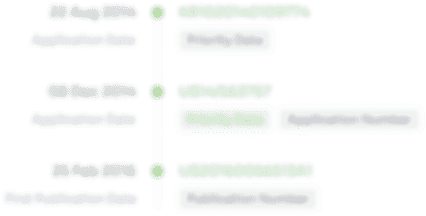
- R&D Engineer
- R&D Manager
- IP Professional
- Industry Leading Data Capabilities
- Powerful AI technology
- Patent DNA Extraction
Browse by: Latest US Patents, China's latest patents, Technical Efficacy Thesaurus, Application Domain, Technology Topic, Popular Technical Reports.
© 2024 PatSnap. All rights reserved.Legal|Privacy policy|Modern Slavery Act Transparency Statement|Sitemap|About US| Contact US: help@patsnap.com