An Image Classification Method Including Online Few-Sample Incentives
A classification method and small sample technology, applied in the field of image processing, can solve problems such as difficulty in producing results, inability to adapt to classification tasks, etc., to achieve the effect of enhancing influence
- Summary
- Abstract
- Description
- Claims
- Application Information
AI Technical Summary
Problems solved by technology
Method used
Image
Examples
Embodiment Construction
[0056] The accompanying drawings are only used to illustrate the present invention, and should not be construed as limitations on the present patent; the technical solutions of the present invention will be further described below with reference to the accompanying drawings.
[0057] An image classification method with online few-shot excitation, including two stages of training and prediction,
[0058] The training data in the training phase includes several life cycles (episodes), and each life cycle includes the following steps:
[0059] The first step is to randomly select 5 categories from all categories in the training set, number them as categories 1-5, and then randomly select a picture from the categories 1-5 to form a set of 5 reference pictures;
[0060] The second step is to randomly select a class from 1-5 categories, and then select a picture in it as a test picture for this life cycle;
[0061] The third step is to perform the following operations on the 5 refe...
PUM
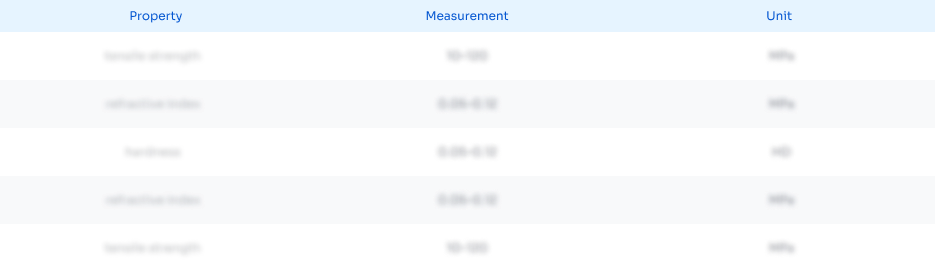
Abstract
Description
Claims
Application Information
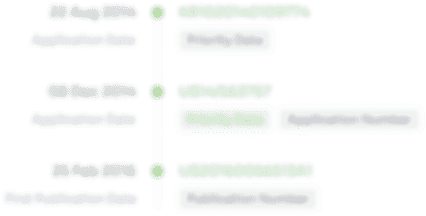
- R&D Engineer
- R&D Manager
- IP Professional
- Industry Leading Data Capabilities
- Powerful AI technology
- Patent DNA Extraction
Browse by: Latest US Patents, China's latest patents, Technical Efficacy Thesaurus, Application Domain, Technology Topic, Popular Technical Reports.
© 2024 PatSnap. All rights reserved.Legal|Privacy policy|Modern Slavery Act Transparency Statement|Sitemap|About US| Contact US: help@patsnap.com