Super-pixel classification method based on semi-supervised K-SVD and multi-scale sparse representation
A technology of sparse representation and classification method, applied in the directions of instrument, character and pattern recognition, scene recognition, etc., can solve problems such as difficulty, single scale of adjacent space area, and assumption of danger
- Summary
- Abstract
- Description
- Claims
- Application Information
AI Technical Summary
Problems solved by technology
Method used
Image
Examples
Embodiment Construction
[0068] The following will clearly and completely describe the technical solutions in the embodiments of the present invention with reference to the accompanying drawings in the embodiments of the present invention. Obviously, the described embodiments are only some, not all, embodiments of the present invention. Based on the embodiments of the present invention, all other embodiments obtained by persons of ordinary skill in the art without making creative efforts belong to the protection scope of the present invention.
[0069] The algorithm in this paper includes two parts: the semi-supervised K-SVD dictionary learning algorithm and the multi-scale sparse representation solution based on superpixels. Firstly, the training samples of hyperspectral images are given to semi-supervised K-SVD dictionary learning to obtain an over-complete dictionary with clear features; then the test samples and over-complete dictionary are given as input to the multi-scale sparse representation al...
PUM
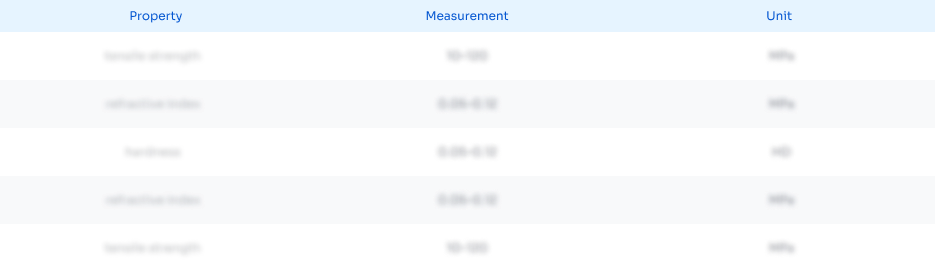
Abstract
Description
Claims
Application Information
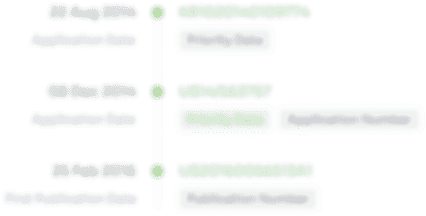
- Generate Ideas
- Intellectual Property
- Life Sciences
- Materials
- Tech Scout
- Unparalleled Data Quality
- Higher Quality Content
- 60% Fewer Hallucinations
Browse by: Latest US Patents, China's latest patents, Technical Efficacy Thesaurus, Application Domain, Technology Topic, Popular Technical Reports.
© 2025 PatSnap. All rights reserved.Legal|Privacy policy|Modern Slavery Act Transparency Statement|Sitemap|About US| Contact US: help@patsnap.com