A text classification method based on a generative multi-task learning model
A multi-task learning and text classification technology, applied in the field of text classification based on a generative multi-task learning model, can solve problems such as difficult comprehensive optimization of classification effects, and achieve the effect of improving classification performance and enhancing semantic association
- Summary
- Abstract
- Description
- Claims
- Application Information
AI Technical Summary
Problems solved by technology
Method used
Image
Examples
Embodiment Construction
[0014] The following will clearly and completely describe the technical solutions in the embodiments of the present invention with reference to the accompanying drawings in the embodiments of the present invention. Obviously, the described embodiments are only some, not all, embodiments of the present invention. Based on the embodiments of the present invention, all other embodiments obtained by persons of ordinary skill in the art without making creative efforts belong to the protection scope of the present invention.
[0015] An embodiment of the present invention provides a text classification method based on a generative multi-task learning model.
[0016] Such as figure 1 As shown, the framework implemented by the present invention includes a data input and preprocessing module, a word embedding module, and an encoding module. The multi-label classification task and the hierarchical classification task have independent attention mechanisms respectively, and include indepe...
PUM
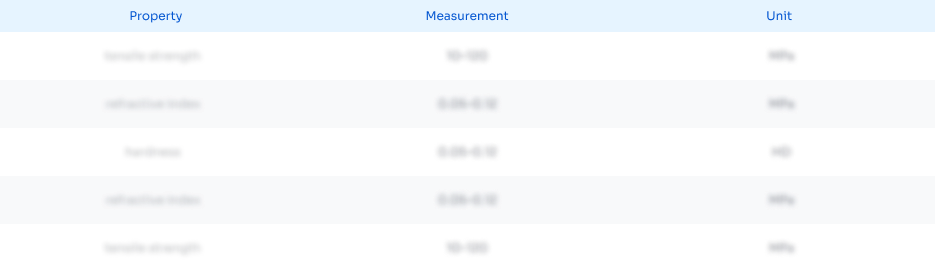
Abstract
Description
Claims
Application Information
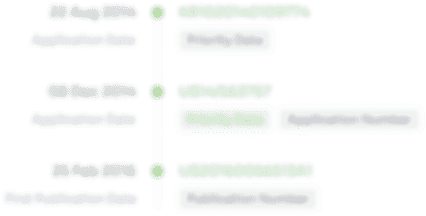
- R&D Engineer
- R&D Manager
- IP Professional
- Industry Leading Data Capabilities
- Powerful AI technology
- Patent DNA Extraction
Browse by: Latest US Patents, China's latest patents, Technical Efficacy Thesaurus, Application Domain, Technology Topic, Popular Technical Reports.
© 2024 PatSnap. All rights reserved.Legal|Privacy policy|Modern Slavery Act Transparency Statement|Sitemap|About US| Contact US: help@patsnap.com