Task unloading method for deep learning application in edge computing environment
An edge computing and deep learning technology, applied in the field of edge computing and deep learning, can solve the problems of ineffective use of terminal computing power, prolonged task execution time, and increased edge load, etc. low-complexity effects
- Summary
- Abstract
- Description
- Claims
- Application Information
AI Technical Summary
Benefits of technology
Problems solved by technology
Method used
Image
Examples
Embodiment Construction
[0058] The technical solutions and beneficial effects of the present invention will be described in detail below in conjunction with the accompanying drawings.
[0059] The present invention provides a task offloading method for deep learning applications in an edge computing environment, which includes four parts, namely building an edge computing execution framework, collecting system information, offloading modeling analysis, and task offloading decision-making. The specific implementation method is as follows:
[0060] In the part of building the edge computing execution framework, cooperate with figure 1 As shown, the present invention combines the idea of deep neural network branch network to build an edge computing execution framework for deep learning applications, including three logical steps of model training, task offloading, and task execution. During model training, the deep neural network is split into three cascadable model blocks, and distributed in differe...
PUM
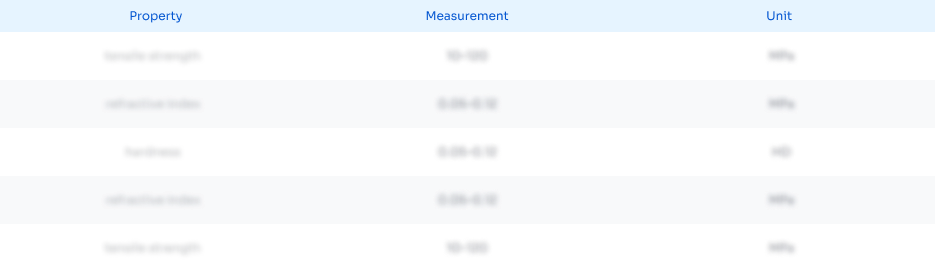
Abstract
Description
Claims
Application Information
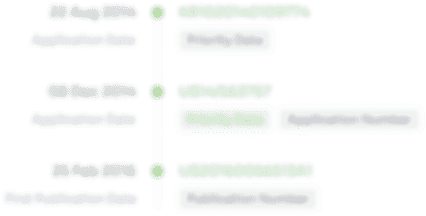
- R&D Engineer
- R&D Manager
- IP Professional
- Industry Leading Data Capabilities
- Powerful AI technology
- Patent DNA Extraction
Browse by: Latest US Patents, China's latest patents, Technical Efficacy Thesaurus, Application Domain, Technology Topic.
© 2024 PatSnap. All rights reserved.Legal|Privacy policy|Modern Slavery Act Transparency Statement|Sitemap