CNN algorithm and Lasso regression model-based hot-rolling product quality prediction method
A regression model and product quality technology, applied in neural learning methods, biological neural network models, calculations, etc., to improve prediction accuracy and alleviate over-fitting effects
- Summary
- Abstract
- Description
- Claims
- Application Information
AI Technical Summary
Problems solved by technology
Method used
Image
Examples
Embodiment Construction
[0060] In order to better explain the present invention and facilitate understanding, the present invention will be described in detail below through specific embodiments in conjunction with the accompanying drawings.
[0061] The invention discloses a method for predicting the quality of hot-rolled products based on CNN algorithm and Lasso regression model, comprising the following steps,
[0062] S1: Obtain sample data for modeling in the historical data of hot-rolled product performance, the sample data includes training data, and determine key input variables;
[0063] S2: Use the key input variables of the sample data to train the CNN to obtain the feature vector model;
[0064] S3: Substituting the key input variables of the training data into the feature vector model to obtain the input variables for substituting into the Lasso regression model;
[0065] S4: Determine the optimal regularization factor of the Lasso regression model, and use the input variables obtained ...
PUM
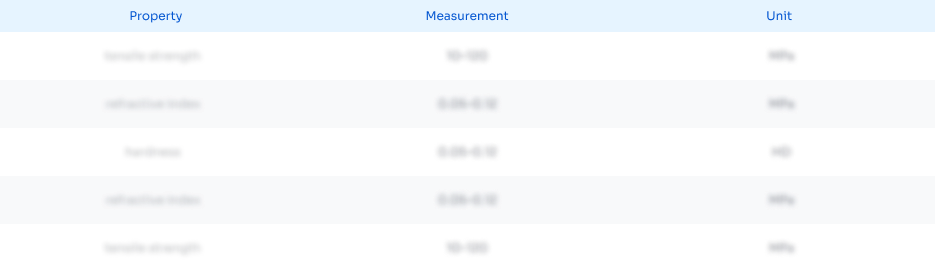
Abstract
Description
Claims
Application Information
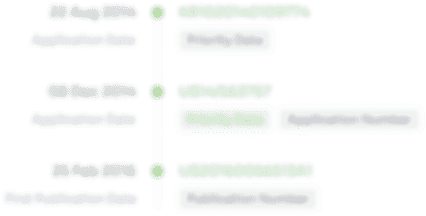
- R&D Engineer
- R&D Manager
- IP Professional
- Industry Leading Data Capabilities
- Powerful AI technology
- Patent DNA Extraction
Browse by: Latest US Patents, China's latest patents, Technical Efficacy Thesaurus, Application Domain, Technology Topic, Popular Technical Reports.
© 2024 PatSnap. All rights reserved.Legal|Privacy policy|Modern Slavery Act Transparency Statement|Sitemap|About US| Contact US: help@patsnap.com