Genetic particle filtering algorithm for interactive multi-model switching and parameter online identification
A particle filter algorithm and interactive multi-model technology, applied in the field of genetic particle filter algorithm, can solve problems such as difficult to meet the requirements of estimation accuracy, deterioration of estimation accuracy, and reduction of estimation accuracy, so as to suppress mutual influence, improve estimation accuracy, very robust effect
- Summary
- Abstract
- Description
- Claims
- Application Information
AI Technical Summary
Problems solved by technology
Method used
Image
Examples
Embodiment Construction
[0020] Such as figure 1 , 2 As shown, a genetic particle filter algorithm for interactive multi-model switching and parameter online identification includes the following steps:
[0021] Step 1: The state estimation method is: use the particle filter algorithm for state estimation, and replace the resampling of the traditional particle filter with the selection, crossover and mutation methods of the genetic algorithm;
[0022] Step 1.1: First, input the state estimation result set at the previous moment, and perform input interactive operation based on the battery model set;
[0023] Step 1.2: Input the measured value of the battery terminal voltage to update the predicted value of the state estimation, and calculate the likelihood function to update the model probability;
[0024] Step 1.3: Finally, according to the model probability calculation results, the state estimation is output and interactively calculated to obtain the final state estimation results;
[0025] Step ...
PUM
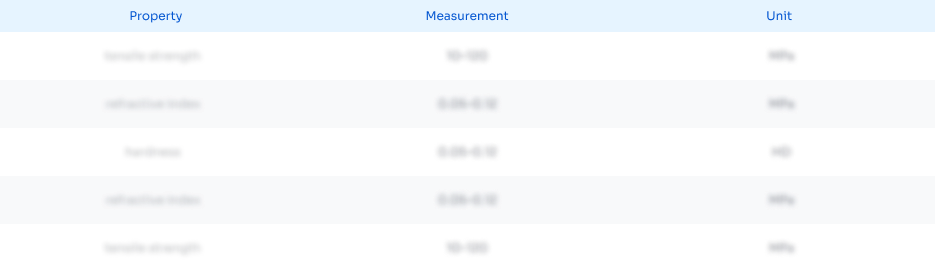
Abstract
Description
Claims
Application Information
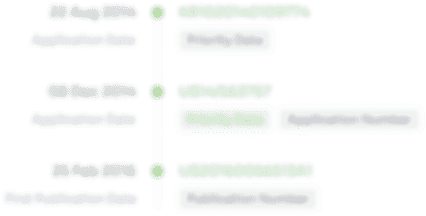
- R&D Engineer
- R&D Manager
- IP Professional
- Industry Leading Data Capabilities
- Powerful AI technology
- Patent DNA Extraction
Browse by: Latest US Patents, China's latest patents, Technical Efficacy Thesaurus, Application Domain, Technology Topic, Popular Technical Reports.
© 2024 PatSnap. All rights reserved.Legal|Privacy policy|Modern Slavery Act Transparency Statement|Sitemap|About US| Contact US: help@patsnap.com