Semi-supervised medical image segmentation method based on adversarial collaborative training
A medical image, collaborative training technology, applied in medical images, healthcare informatics, instruments, etc., can solve problems such as limited pre-training model performance, achieve good universality and versatility, and improve segmentation performance.
- Summary
- Abstract
- Description
- Claims
- Application Information
AI Technical Summary
Problems solved by technology
Method used
Image
Examples
Embodiment Construction
[0041] Below in conjunction with specific embodiment, further illustrate the present invention, should be understood that these embodiments are only used to illustrate the present invention and are not intended to limit the scope of the present invention, after having read the present invention, those skilled in the art will understand various equivalent forms of the present invention All modifications fall within the scope defined by the appended claims of the present application.
[0042] A semi-supervised segmentation method for medical images based on adversarial collaborative training. The schematic diagram of the network structure is as follows: figure 1shown. The network consists of two sub-networks: a segmentation network and a discriminator. The split network uses two encoder-decoder architectures and shares their encoder parts. The two decoder branches can use a co-training approach to supervise each other to improve performance. The discriminator uses a conventio...
PUM
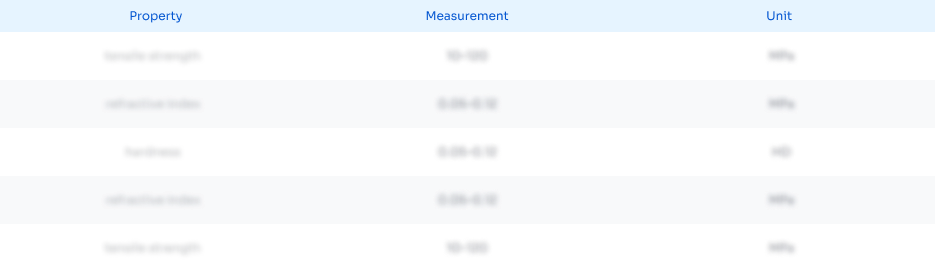
Abstract
Description
Claims
Application Information
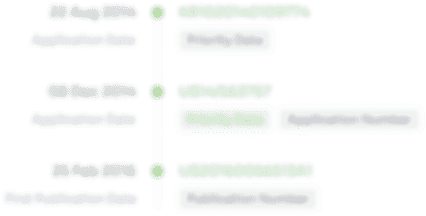
- R&D Engineer
- R&D Manager
- IP Professional
- Industry Leading Data Capabilities
- Powerful AI technology
- Patent DNA Extraction
Browse by: Latest US Patents, China's latest patents, Technical Efficacy Thesaurus, Application Domain, Technology Topic, Popular Technical Reports.
© 2024 PatSnap. All rights reserved.Legal|Privacy policy|Modern Slavery Act Transparency Statement|Sitemap|About US| Contact US: help@patsnap.com