Text TF-IDF feature reconstruction method combined with emotion intensity
A technology of TF-IDF and emotional intensity, which is applied in the classification field in natural language processing, can solve problems such as information confusion, information inaccuracy, and failure to retain, and achieve the effect of avoiding information confusion
- Summary
- Abstract
- Description
- Claims
- Application Information
AI Technical Summary
Problems solved by technology
Method used
Image
Examples
Embodiment
[0044] A text TF-IDF feature reconstruction method combined with emotional strength, first constructing a disabling dictionary, a degree dictionary and a negative dictionary. Stop words mainly include English characters, numbers, mathematical characters, punctuation marks, and single Chinese characters with high frequency, such as "you", "I", "because", "and" and so on; the degree dictionary includes a series of used The adverbs that modify the intensity of adjectives and adverbs and their corresponding emotional intensity, select the "Dictionary of Degree Level Words" released by HowNet in 2007 as the degree dictionary, and divide the degree adverbs into "extremely" , "Super", "Very", "Compare", "Slightly", and "Under", corresponding to six levels of 1.7, 1.5, 1.3, 1.1, 0.8 and 0.5 respectively; the negative dictionary contains "not", Common negative words such as "no", "non" and "weiwei". Such as figure 1 As shown, this method includes the following processes:
[0045] 1....
example 1
[0086] Example 1: @今天also like miuky you look so good today [happy][happy]
[0087]
[0088]
[0089] Compared with the traditional method, the user name "@今天also like miuky" and the expression "[happy]" are correctly segmented.
example 2
[0090] Example 2: I don't like driving
[0091] method Feature vector traditional method Drive: 0.89 Likes: 0.45 The method proposed by the present invention Like: -0.46 Drive: -0.89
[0092] Compared with the traditional method, the reconstructed feature vector preserves the information of the negative word "no".
PUM
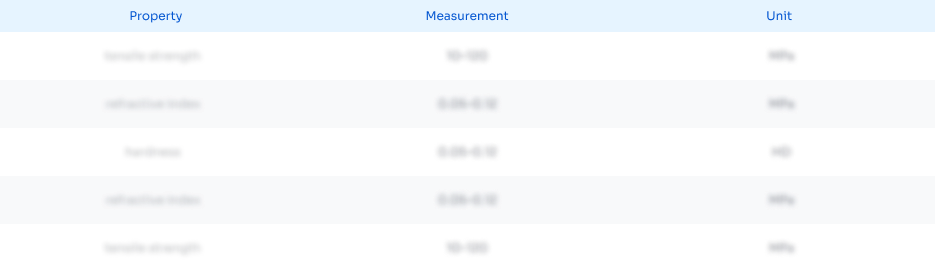
Abstract
Description
Claims
Application Information
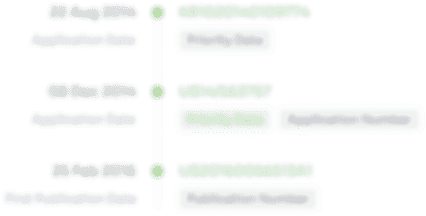
- R&D
- Intellectual Property
- Life Sciences
- Materials
- Tech Scout
- Unparalleled Data Quality
- Higher Quality Content
- 60% Fewer Hallucinations
Browse by: Latest US Patents, China's latest patents, Technical Efficacy Thesaurus, Application Domain, Technology Topic, Popular Technical Reports.
© 2025 PatSnap. All rights reserved.Legal|Privacy policy|Modern Slavery Act Transparency Statement|Sitemap|About US| Contact US: help@patsnap.com