A multi-target tracking method and system based on depth conditional random field model
A conditional random field, multi-target tracking technology, applied in image analysis, image enhancement, instrumentation, etc., can solve the problems of combining inference algorithms with neural networks, reducing the accuracy of target tracking results, and difficulty in solving CRF model parameters.
- Summary
- Abstract
- Description
- Claims
- Application Information
AI Technical Summary
Problems solved by technology
Method used
Image
Examples
Embodiment Construction
[0096] In order to make the objectives, technical solutions and advantages of the present invention clearer, the following further describes the present invention in detail with reference to the accompanying drawings and embodiments. It should be understood that the specific embodiments described herein are only used to explain the present invention, but not to limit the present invention. In addition, the technical features involved in the various embodiments of the present invention described below can be combined with each other as long as they do not conflict with each other.
[0097] The present invention proposes a Conditional Random Fields (CRF, CRF) framework for multi-target tracking. Compared with the existing CRF-based target tracking method, the present invention focuses on the learning of CRF potential energy And difficult CRF reasoning. The neutral network is specially designed to learn more suitable CRF potential energy, and the gradient descent algorithm is used ...
PUM
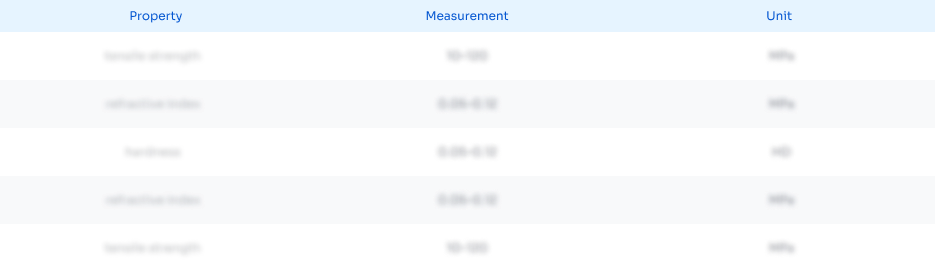
Abstract
Description
Claims
Application Information
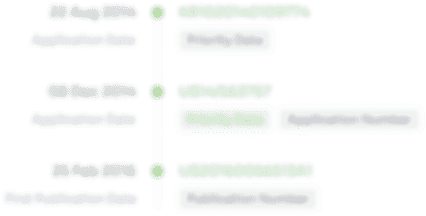
- Generate Ideas
- Intellectual Property
- Life Sciences
- Materials
- Tech Scout
- Unparalleled Data Quality
- Higher Quality Content
- 60% Fewer Hallucinations
Browse by: Latest US Patents, China's latest patents, Technical Efficacy Thesaurus, Application Domain, Technology Topic, Popular Technical Reports.
© 2025 PatSnap. All rights reserved.Legal|Privacy policy|Modern Slavery Act Transparency Statement|Sitemap|About US| Contact US: help@patsnap.com