Traffic data restoration method based on generative adversarial network
A technology of traffic data and repair method, which is applied to data error detection, electrical digital data processing, and response error generation in the direction of redundancy in computing, and can solve problems such as performance degradation
- Summary
- Abstract
- Description
- Claims
- Application Information
AI Technical Summary
Problems solved by technology
Method used
Image
Examples
Embodiment Construction
[0033] In the actual production environment, the recording equipment often loses the recording information of some periods, that is, the complete traffic flow matrix (a) in the upper left corner is missing under natural conditions to form the missing matrix (b). We input the missing matrix (b) into the trained generation network to obtain the repair matrix (c), and add the identification network to train the generation network to ensure the accuracy of the repair results. figure 1 middle x ij is the record of complete data, p ij Is the repaired record, ? is the missing record.
[0034] figure 2 Show the specific structure of the trained model. We feed the missing traffic flow matrix into the generative network, first extract features through convolutional and dilated convolutional layers, and then output the inpainting matrix through convolutional and inverse convolutional layers. After the repair, the traffic matrix is judged by the discriminator network to determine ...
PUM
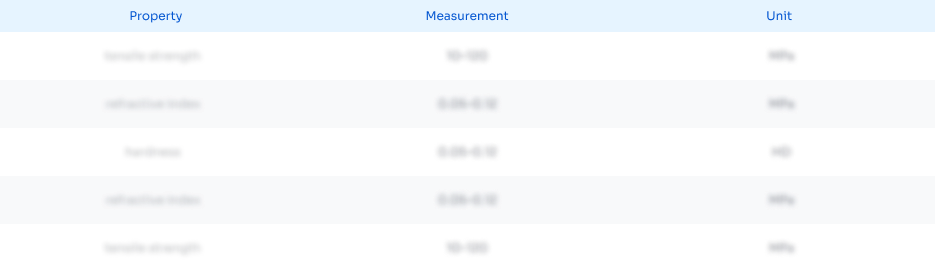
Abstract
Description
Claims
Application Information
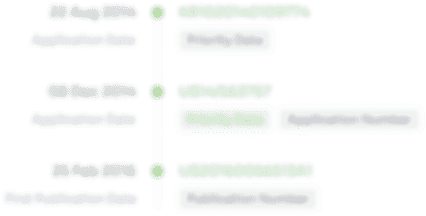
- R&D Engineer
- R&D Manager
- IP Professional
- Industry Leading Data Capabilities
- Powerful AI technology
- Patent DNA Extraction
Browse by: Latest US Patents, China's latest patents, Technical Efficacy Thesaurus, Application Domain, Technology Topic, Popular Technical Reports.
© 2024 PatSnap. All rights reserved.Legal|Privacy policy|Modern Slavery Act Transparency Statement|Sitemap|About US| Contact US: help@patsnap.com