Turbine vibration fault diagnosis method based on deep learning artificial neural network
An artificial neural network and deep learning technology, applied in the field of steam turbine vibration fault diagnosis based on deep learning artificial neural network, can solve problems such as deficiencies
- Summary
- Abstract
- Description
- Claims
- Application Information
AI Technical Summary
Problems solved by technology
Method used
Image
Examples
Embodiment Construction
[0055] figure 1 It is a flow chart of the steam turbine fault diagnosis method based on the deep learning artificial neural network of the present invention. The actual working parameters of the steam turbine unit are measured by sensors, and then various vibration faults and parameter sets when faults occur are established as training samples.
[0056] The fault data uses the data simulated by the simulation signal, and the parameter sampling frequency is 100k. Extract vibration features, non-vibration features and running status labels from the input samples to form a data sample set.
[0057] The data sample set has a total of 15,000 samples, each of which contains 10 types of steam turbine parameters and 1 operating status label. There are 13 common fault operating status labels and 1 normal operating status label. Samples with normal running labels are called normal samples, and samples with faulty running labels are called faulty samples. Each fault operating state co...
PUM
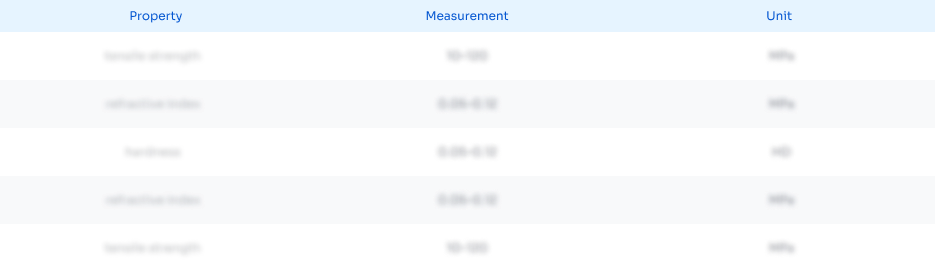
Abstract
Description
Claims
Application Information
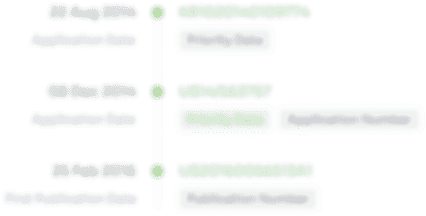
- R&D Engineer
- R&D Manager
- IP Professional
- Industry Leading Data Capabilities
- Powerful AI technology
- Patent DNA Extraction
Browse by: Latest US Patents, China's latest patents, Technical Efficacy Thesaurus, Application Domain, Technology Topic, Popular Technical Reports.
© 2024 PatSnap. All rights reserved.Legal|Privacy policy|Modern Slavery Act Transparency Statement|Sitemap|About US| Contact US: help@patsnap.com