An image compressed sensing reconstruction method based on a non-local self-similarity model
An image compression, non-local technology, applied in image coding, image data processing, computer components, etc., can solve the problems of data calculation and memory resource waste, poor CS effect, and inaccurate sparse coding, etc.
- Summary
- Abstract
- Description
- Claims
- Application Information
AI Technical Summary
Problems solved by technology
Method used
Image
Examples
Embodiment Construction
[0021] specific implementation
[0022] 1. Construct the regular term of the non-local self-similar model of the image. In the field of image processing, one of the most important characteristics of natural image signals is non-local self-similarity, that is, an image block has many similar blocks in other positions in the image, and the structural information of the image is redundant. Using this property can Better describe the texture and details of the image, and then improve the reconstruction effect of the image. A priori model of natural images based on the features of repetitive structural patterns contained in the image itself
[0023] Such as figure 2 Shown in: in the image block x 0 Similar blocks exist in the neighborhood of : Therefore image block x 0 It can be weighted by similar blocks in the neighborhood:
[0024]
[0025] in is the weight, so for the entire image block:
[0026]
[0027] where j represents the number of similar blocks, so that...
PUM
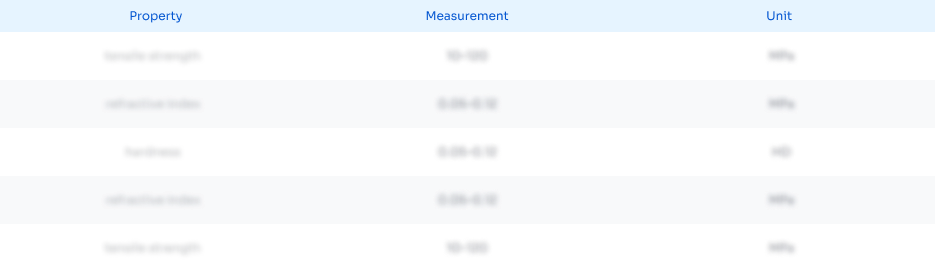
Abstract
Description
Claims
Application Information
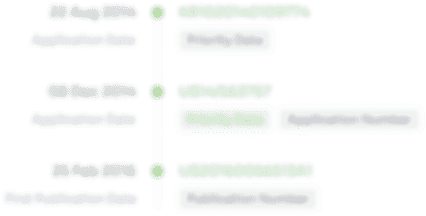
- R&D Engineer
- R&D Manager
- IP Professional
- Industry Leading Data Capabilities
- Powerful AI technology
- Patent DNA Extraction
Browse by: Latest US Patents, China's latest patents, Technical Efficacy Thesaurus, Application Domain, Technology Topic, Popular Technical Reports.
© 2024 PatSnap. All rights reserved.Legal|Privacy policy|Modern Slavery Act Transparency Statement|Sitemap|About US| Contact US: help@patsnap.com