An Unsupervised Image Recognition Method Based on Parameter Transfer Learning
A transfer learning and image recognition technology, applied in the field of image recognition, can solve the problems of long training time and large number of unlabeled samples, reducing training time, solving unsupervised recognition problems, and improving learning efficiency.
- Summary
- Abstract
- Description
- Claims
- Application Information
AI Technical Summary
Problems solved by technology
Method used
Image
Examples
specific Embodiment approach 1
[0035] Specific implementation mode one: as figure 1 As shown, an unsupervised image recognition method based on parameter transfer learning described in this embodiment, the method includes the following steps:
[0036] Step 1. Collect images containing category labels from the auxiliary domain to form an auxiliary domain image set X s ;Collect images without class labels from the application domain to form the application domain image set X t ; The application field refers to various fields that the method of the present invention can be applied to, and the auxiliary field refers to a field where the sample content is similar to the field to be applied and contains a large number of tags;
[0037] Step 2. Construct two convolutional neural networks with the same structure, and use the two convolutional neural networks with the same structure as the auxiliary domain network and the application domain network respectively, where: the auxiliary domain network is recorded as N ...
specific Embodiment approach 2
[0048] Specific implementation mode two: the difference between this implementation mode and specific implementation mode one is: the specific process of the step one is:
[0049] Collect images containing category labels from the auxiliary domain to form an auxiliary domain image set X s ;Collect images without class labels from the application domain to form the application domain image set X t ; where: application domain image set X t The number of image samples in is the auxiliary domain image set X s One-tenth of the number of image samples in ;
[0050] The auxiliary domain image set X s with application domain image set X t All images in are scaled to the same size.
specific Embodiment approach 3
[0051] Specific implementation mode three: the difference between this implementation mode and specific implementation mode one is: the specific process of the step two is:
[0052] Construct two convolutional neural networks with the same structure, and use the two convolutional neural networks with the same structure as the auxiliary domain network and the application domain network respectively, where: the auxiliary domain network is recorded as N s , the application domain network is denoted as N t ;
[0053] Such as figure 2 As shown, each convolutional neural network includes five layers of convolutional layers conv1~conv5 and three layers of fully connected layers fc1~fc3, wherein: the fully connected layer is located after the convolutional layer;
[0054] After the fully connected layer is an image classifier, the image classifier has a total of C branches, where: C represents the total number of image categories that can be recognized; and the output y of the cth ...
PUM
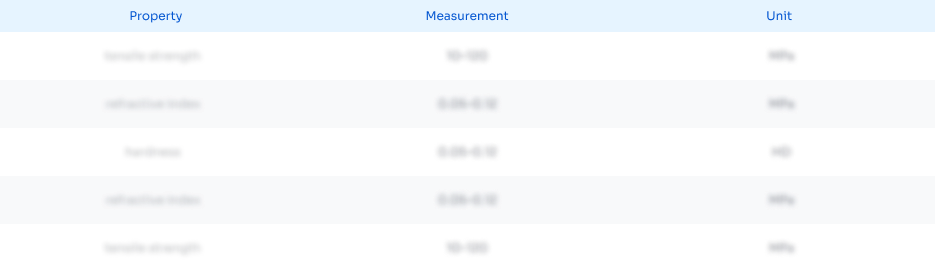
Abstract
Description
Claims
Application Information
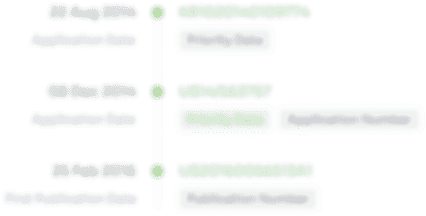
- Generate Ideas
- Intellectual Property
- Life Sciences
- Materials
- Tech Scout
- Unparalleled Data Quality
- Higher Quality Content
- 60% Fewer Hallucinations
Browse by: Latest US Patents, China's latest patents, Technical Efficacy Thesaurus, Application Domain, Technology Topic, Popular Technical Reports.
© 2025 PatSnap. All rights reserved.Legal|Privacy policy|Modern Slavery Act Transparency Statement|Sitemap|About US| Contact US: help@patsnap.com