A method and apparatus for training a model based on a gradient lifting decision tree
A decision tree and gradient technology, applied in the information field, can solve problems such as inability to train qualified ones and less data
- Summary
- Abstract
- Description
- Claims
- Application Information
AI Technical Summary
Problems solved by technology
Method used
Image
Examples
Embodiment Construction
[0037] The invention draws on the transfer learning idea in the field of machine learning technology. When faced with the need to train a model applied to the target business scenario, if the data accumulated in the target business scenario is insufficient, then the data accumulated in the business scenario similar to the target business scenario can be used for model training.
[0038] Specifically, the present invention combines the migration learning idea with the gradient boosting decision tree (Gradient Boosting Decision Tree, GBDT) algorithm to improve the GBDT algorithm flow. In the embodiment of this specification, for a GBDT algorithm process, first use data generated in a business scenario similar to the target business scenario for training, and after meeting certain training suspension conditions, suspend training and calculate the current training residual; then , Use the data generated in the target business scenario, and continue training based on the training resi...
PUM
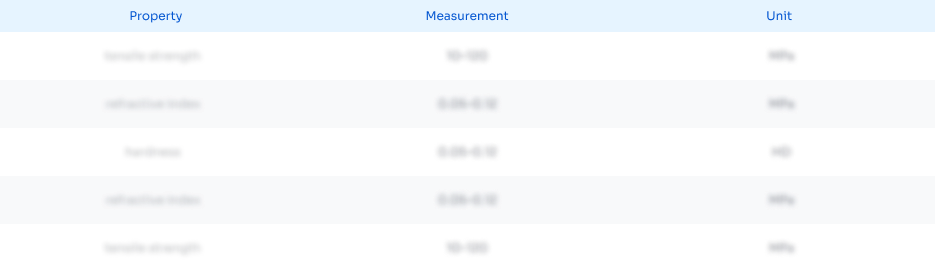
Abstract
Description
Claims
Application Information
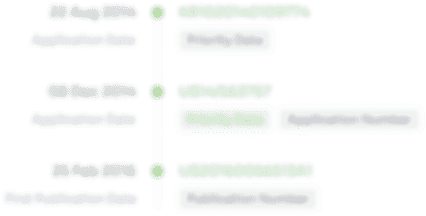
- Generate Ideas
- Intellectual Property
- Life Sciences
- Materials
- Tech Scout
- Unparalleled Data Quality
- Higher Quality Content
- 60% Fewer Hallucinations
Browse by: Latest US Patents, China's latest patents, Technical Efficacy Thesaurus, Application Domain, Technology Topic, Popular Technical Reports.
© 2025 PatSnap. All rights reserved.Legal|Privacy policy|Modern Slavery Act Transparency Statement|Sitemap|About US| Contact US: help@patsnap.com