A Multi-step Forecasting Method for Chaotic Time Series Based on Density Peak Clustering
A chaotic time series, multi-step forecasting technology, applied in forecasting, data processing applications, instruments, etc., can solve the problems of high dependence on forecast starting point and low reliability, achieve good practical use value, less training set, and improve forecasting The effect of precision
- Summary
- Abstract
- Description
- Claims
- Application Information
AI Technical Summary
Problems solved by technology
Method used
Image
Examples
Embodiment Construction
[0041] According to the phase space reconstruction theory, the present invention reconstructs the chaotic time series to obtain the phase point training set, and then screens out the effective phase points that can describe the next change of the time series, and uses them as filter training to obtain the prediction of the chaotic time series value. Concrete steps of the present invention are as follows:
[0042] Step 1. For the chaotic time series, use the delay coordinate method to reconstruct the phase space to obtain the phase point training set composed of phase points; then cluster the phase point training set to obtain the clustered training set.
[0043]In this embodiment, because too many clustering centers not only affect the clustering efficiency, but also bring obstacles in the process of screening the nearest orbits, after experiments, it is found that 5 clustering centers are more suitable; the clustering in this embodiment The algorithm is as follows:
[0044]...
PUM
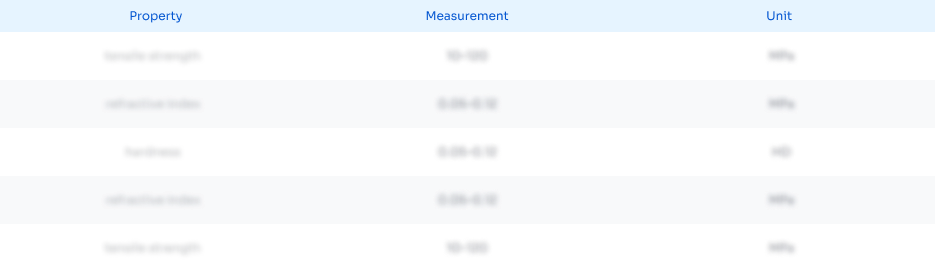
Abstract
Description
Claims
Application Information
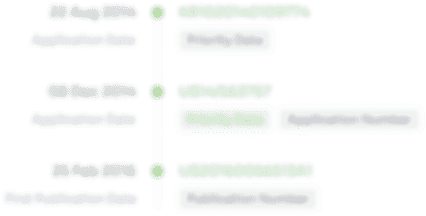
- R&D Engineer
- R&D Manager
- IP Professional
- Industry Leading Data Capabilities
- Powerful AI technology
- Patent DNA Extraction
Browse by: Latest US Patents, China's latest patents, Technical Efficacy Thesaurus, Application Domain, Technology Topic, Popular Technical Reports.
© 2024 PatSnap. All rights reserved.Legal|Privacy policy|Modern Slavery Act Transparency Statement|Sitemap|About US| Contact US: help@patsnap.com