Global optimization system and method based on continuous motion learning automata
A technology of global optimization and action learning, applied in machine learning, computational models, computing, etc., can solve the problems of local minima and weak anti-noise ability, and achieve the effect of improving the convergence speed
- Summary
- Abstract
- Description
- Claims
- Application Information
AI Technical Summary
Problems solved by technology
Method used
Image
Examples
Embodiment Construction
[0024] In the field of optimal path selection and optimal treatment effect (such as: maximization of information dissemination, optimal metering drug selection, etc.), this method can first obtain the local optimal solution of the current path through the existing CALA algorithm, because the path It may be only a local optimal path, not a global optimal path, and then an improved smoothing function is obtained according to the local optimal solution; the improved smoothing function is introduced into the existing CALA algorithm to obtain an optimized CALA algorithm, and the optimized CALA algorithm is used to perform After multiple iterations, the extreme point is finally obtained, which is output as the global minimum value after path selection, so as to obtain the optimal path selection scheme.
[0025] Described iteration refers to: introduce improved smoothing function in CALA algorithm and carry out one round of iteration to obtain local optimum solution, when this round o...
PUM
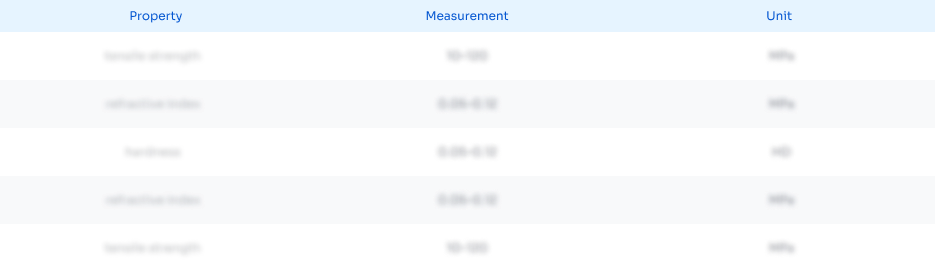
Abstract
Description
Claims
Application Information
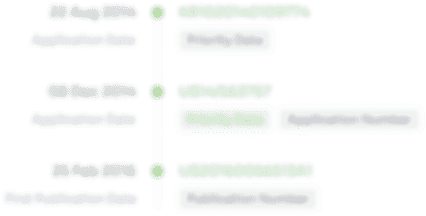
- R&D Engineer
- R&D Manager
- IP Professional
- Industry Leading Data Capabilities
- Powerful AI technology
- Patent DNA Extraction
Browse by: Latest US Patents, China's latest patents, Technical Efficacy Thesaurus, Application Domain, Technology Topic, Popular Technical Reports.
© 2024 PatSnap. All rights reserved.Legal|Privacy policy|Modern Slavery Act Transparency Statement|Sitemap|About US| Contact US: help@patsnap.com