KF (Kalman Filter) tracking method based on fading memory exponential weighting
A Kalman filtering and exponential weighting technology, which is applied in the direction of navigation, measuring devices, instruments, etc. through velocity/acceleration measurement, can solve the problem of large calculation errors of fading factors, reduce the accuracy of algorithm estimation, and reduce the correction effect of innovation covariance matrix And other issues
- Summary
- Abstract
- Description
- Claims
- Application Information
AI Technical Summary
Problems solved by technology
Method used
Image
Examples
Embodiment 1
[0108] Embodiment one validity analysis
[0109] In order to analyze the effectiveness of the method in this paper, the filter estimation is first carried out in the case of matching the statistical characteristics of the noise, and the data in the simulation experiment is set to R k =Q k =diag[0.01 0.01 0.01 0.01], and the setting is also considered to be satisfied during the simulation process, and the filtering tracking results are as follows figure 1 and figure 2 shown. From figure 1 and figure 2 It can be seen from the figure that in the case of noise matching, the three methods can achieve good estimation, and the overall errors of the three methods are all controlled within 0.04, which can meet the high-precision estimation requirements, and verify the correction of the method in this paper. It's effective. Further, from figure 2 It can be seen that the estimation accuracy of the method in this paper and the fading factor KF method is slightly better than that...
Embodiment 2
[0110] Embodiment two superiority analysis
[0111] In order to analyze the superiority of the method estimated in this paper in the case of mismatch, the noise parameters of the actual data are amplified by 10 times and 50 times respectively, but in the actual algorithm parameters, the noise characteristics are still considered to meet the standard of formula (26), and the noise algorithm is the same as the actual The noise statistical characteristics of the environment differ by 10 times, and the filtering and tracking results are as follows: image 3 to map Figure 6 shown.
[0112] From image 3 and Figure 4 It can be seen from the figure that when the state noise mismatch is 10 times, both the fading factor KF method and the method in this paper maintain a high estimation accuracy, and the absolute value of the estimation error is controlled within 0.04m, while the estimation error of KF The absolute value exceeds 0.04m. It shows that the method in this paper and th...
PUM
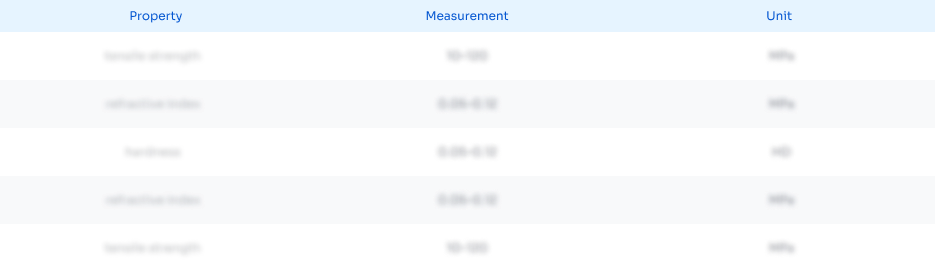
Abstract
Description
Claims
Application Information
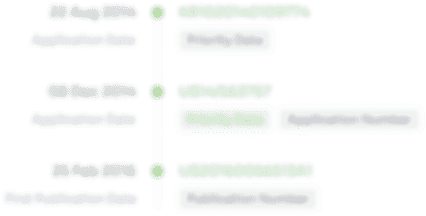
- R&D Engineer
- R&D Manager
- IP Professional
- Industry Leading Data Capabilities
- Powerful AI technology
- Patent DNA Extraction
Browse by: Latest US Patents, China's latest patents, Technical Efficacy Thesaurus, Application Domain, Technology Topic, Popular Technical Reports.
© 2024 PatSnap. All rights reserved.Legal|Privacy policy|Modern Slavery Act Transparency Statement|Sitemap|About US| Contact US: help@patsnap.com