Image semantic segmentation method
A semantic segmentation and image technology, applied in the field of image semantic segmentation using deep learning, can solve the problems of reduced resolution of feature maps, insensitivity to details, and unfavorable dense prediction tasks.
- Summary
- Abstract
- Description
- Claims
- Application Information
AI Technical Summary
Problems solved by technology
Method used
Image
Examples
Embodiment Construction
[0029] The present invention will be described in further detail below in conjunction with the accompanying drawings.
[0030] The designer of the present invention has devoted himself to the research of semantic image segmentation, summed up and aimed at the deficiencies and drawbacks of the current existing technology, and innovatively proposed a brand-new semantic image segmentation method. By making some improvements in the structure of U-net, the design A novel encoder-decoder structure, called dense deconvolution network (Dense Deconvolution Network, DDN). Compared with other networks, this network can better take into account local and global information. The shape and boundary of objects in the final segmentation map are clearer, the classification is more accurate, and the resolution of the segmentation map is the same as that of the original image.
[0031] figure 1 It is a detailed structural diagram of the present invention, which mainly includes two parts: convol...
PUM
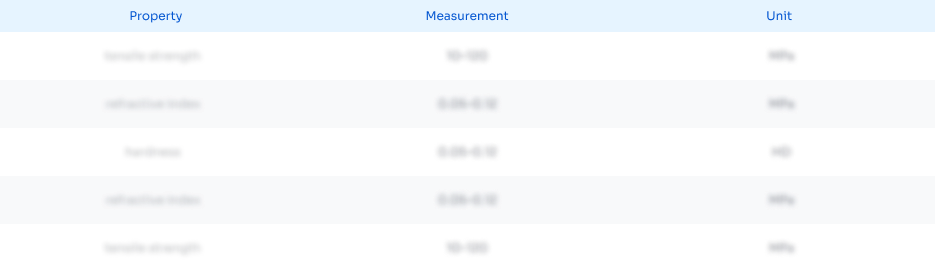
Abstract
Description
Claims
Application Information
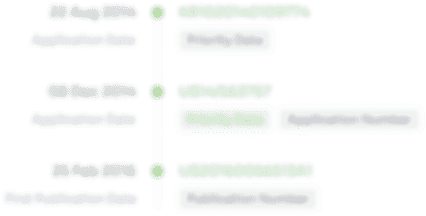
- R&D Engineer
- R&D Manager
- IP Professional
- Industry Leading Data Capabilities
- Powerful AI technology
- Patent DNA Extraction
Browse by: Latest US Patents, China's latest patents, Technical Efficacy Thesaurus, Application Domain, Technology Topic, Popular Technical Reports.
© 2024 PatSnap. All rights reserved.Legal|Privacy policy|Modern Slavery Act Transparency Statement|Sitemap|About US| Contact US: help@patsnap.com