A superimposed velocity spectrum picking method and processing terminal based on deep reinforcement learning
A technology of superimposed velocity spectrum and reinforcement learning, which is applied in the field of superimposed velocity spectrum picking method and processing terminal based on deep reinforcement learning, which can solve the problems of complex operation, low efficiency, difficult identification and tracking, etc.
- Summary
- Abstract
- Description
- Claims
- Application Information
AI Technical Summary
Problems solved by technology
Method used
Image
Examples
Embodiment Construction
[0063] Below, in conjunction with accompanying drawing and specific embodiment, the present invention is described further:
[0064] Such as figure 1 As shown, this embodiment discloses a method for picking up a superimposed velocity spectrum based on deep reinforcement learning, which includes the following steps:
[0065] Step S1: Obtain the original common-centroid seismic gather data including seismic reflection waves;
[0066] Step S2: According to the common center point seismic gather data in step S1, the stacking velocity spectrum obtained by scanning the scanning speed at each time is calculated by the preset algorithm. The stacking velocity spectrum is composed of the optimal scanning speed, and the output is two-dimensional array representation of the stacked velocity spectrum;
[0067] Specifically, the calculation of the stacked velocity spectrum is performed in the following steps:
[0068] a. Put the seismic reflection wave in step S1 into the corresponding p...
PUM
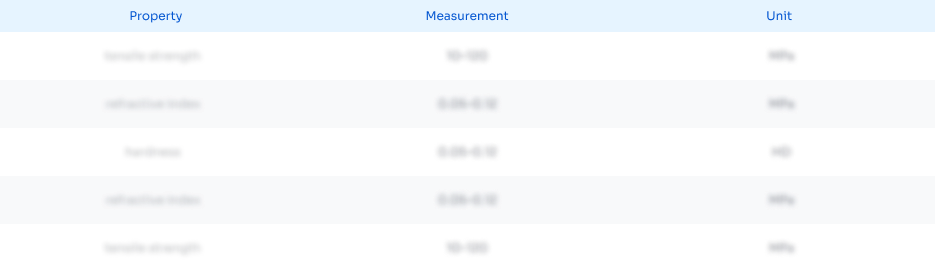
Abstract
Description
Claims
Application Information
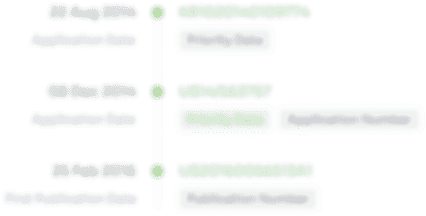
- R&D Engineer
- R&D Manager
- IP Professional
- Industry Leading Data Capabilities
- Powerful AI technology
- Patent DNA Extraction
Browse by: Latest US Patents, China's latest patents, Technical Efficacy Thesaurus, Application Domain, Technology Topic, Popular Technical Reports.
© 2024 PatSnap. All rights reserved.Legal|Privacy policy|Modern Slavery Act Transparency Statement|Sitemap|About US| Contact US: help@patsnap.com