Polarized SAR Image Classification Method Based on Residual Learning and Conditional GAN
A residual and image technology, applied in the field of polarization synthetic aperture radar SAR image classification, can solve the problems of incomplete slice feature context information, poor regional consistency, low classification accuracy, etc., achieve good regional consistency, improve classification Accuracy, reducing the effect of small image spots
- Summary
- Abstract
- Description
- Claims
- Application Information
AI Technical Summary
Problems solved by technology
Method used
Image
Examples
Embodiment Construction
[0047] The present invention will be described in further detail below in conjunction with the accompanying drawings.
[0048] refer to figure 1 , to further describe in detail the implementation steps of the present invention.
[0049] Step 1. Construct the generator of conditional generative adversarial network GAN.
[0050] Build a 27-layer conditional generation against the network GAN generator, its structure is as follows: input layer → first convolutional layer → second convolutional layer → first pixel addition layer → pooling layer → third convolutional layer →First upsampling layer→Second pixel addition layer→Pooling layer→Fourth convolutional layer→Second upsampling layer→Third pixel addition layer→Pooling layer→Fifth convolutional layer→Third upper Sampling layer → fourth pixel addition layer → fourth upsampling layer → sixth convolutional layer → fifth upsampling layer → fifth pixel addition layer → sixth upsampling layer → seventh convolutional layer → seventh ...
PUM
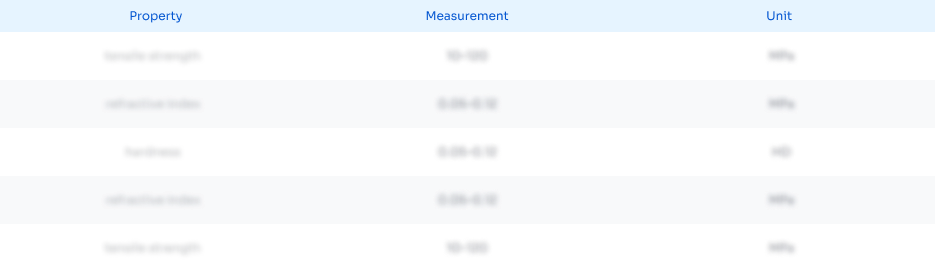
Abstract
Description
Claims
Application Information
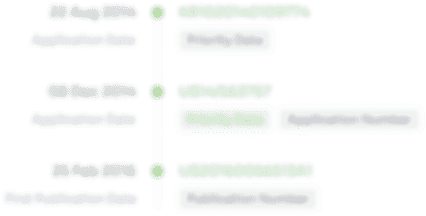
- R&D Engineer
- R&D Manager
- IP Professional
- Industry Leading Data Capabilities
- Powerful AI technology
- Patent DNA Extraction
Browse by: Latest US Patents, China's latest patents, Technical Efficacy Thesaurus, Application Domain, Technology Topic, Popular Technical Reports.
© 2024 PatSnap. All rights reserved.Legal|Privacy policy|Modern Slavery Act Transparency Statement|Sitemap|About US| Contact US: help@patsnap.com