Vector space computing strength predicting method and system based on fully random forest
A technology for calculating intensity and vector space, which is applied in calculation, still image data in vector format, design optimization/simulation, etc. It can solve the problems that have not been considered, cannot deal with the interaction of different features, and it is difficult to achieve accurate measurement of vector space calculation intensity. Achieve the effect of improving prediction accuracy and improving parallel computing efficiency
- Summary
- Abstract
- Description
- Claims
- Application Information
AI Technical Summary
Problems solved by technology
Method used
Image
Examples
Embodiment Construction
[0024] In order to have a clearer understanding of the technical features, purposes and effects of the present invention, the specific implementation manners of the present invention will now be described in detail with reference to the accompanying drawings.
[0025] Such as figure 1 as shown, figure 1 It is a flow chart of the vector space calculation intensity prediction method based on complete random forest provided by the present invention, and the method includes the following steps:
[0026] Step 1) Obtain the original sample set of vector space calculation intensity, each vector space calculation intensity sample includes 13 types of values, which are respectively the number of elements contained in the vector layer, element geometry type, database type, and layer space range Width, layer spatial range height, number of elements in the spatial calculation domain, number of vertices in the spatial calculation domain, window pixel width, window pixel height, window geo...
PUM
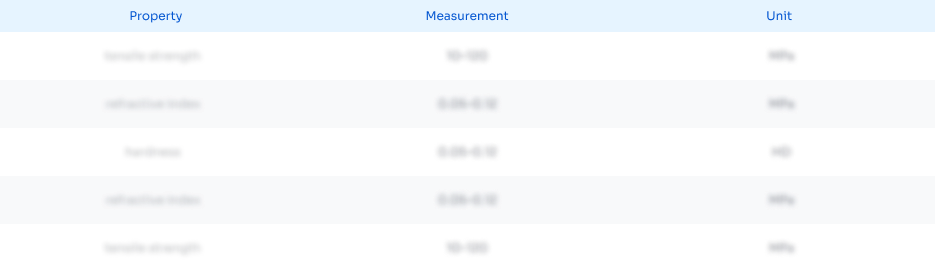
Abstract
Description
Claims
Application Information
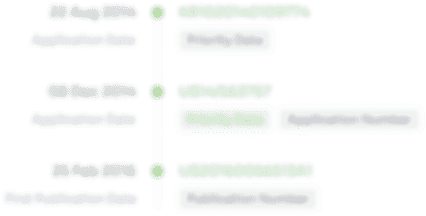
- R&D Engineer
- R&D Manager
- IP Professional
- Industry Leading Data Capabilities
- Powerful AI technology
- Patent DNA Extraction
Browse by: Latest US Patents, China's latest patents, Technical Efficacy Thesaurus, Application Domain, Technology Topic, Popular Technical Reports.
© 2024 PatSnap. All rights reserved.Legal|Privacy policy|Modern Slavery Act Transparency Statement|Sitemap|About US| Contact US: help@patsnap.com