Cross-modal retrieval method based on deep correlation network
An associative network and cross-modal technology, applied in biological neural network models, multimedia data retrieval, special data processing applications, etc., can solve the problems of unstable retrieval effect, single types of deep network components, and low retrieval accuracy, and achieve Good performance, high precision, good stability
- Summary
- Abstract
- Description
- Claims
- Application Information
AI Technical Summary
Problems solved by technology
Method used
Image
Examples
Embodiment
[0106] Assume that we have o pairs of text and image data with known correspondence, that is, training set data; k text data and image data with unknown correspondence, that is, test set data; take image retrieval text as an example to illustrate, then retrieve The target is an image s in the test set, and the retrieval library contains k retrieval members in the test set, and the retrieval members are all text modal data; for example Figure 7 shown, including the following three steps:
[0107] 1) Step 701: use the initial feature method to extract the features of the text and image data of the known correspondence in the training set o to form a primary vector, and extract the features of k in the test set to the text data and image data of the unknown correspondence to form a primary vector;
[0108] The original data of different modalities has its mature initial feature extraction method; the retrieval target is the data of the image modality, and the data of the image m...
PUM
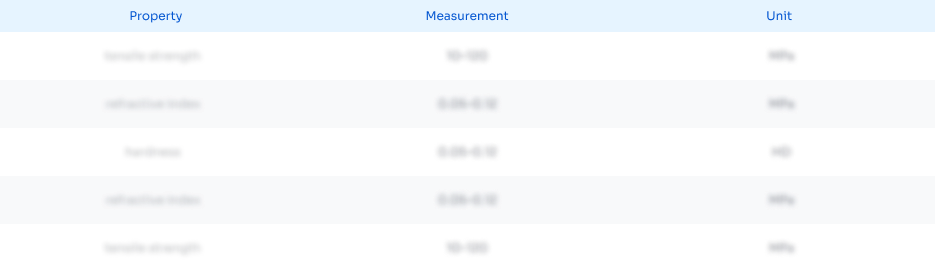
Abstract
Description
Claims
Application Information
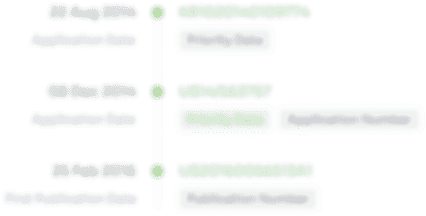
- R&D Engineer
- R&D Manager
- IP Professional
- Industry Leading Data Capabilities
- Powerful AI technology
- Patent DNA Extraction
Browse by: Latest US Patents, China's latest patents, Technical Efficacy Thesaurus, Application Domain, Technology Topic, Popular Technical Reports.
© 2024 PatSnap. All rights reserved.Legal|Privacy policy|Modern Slavery Act Transparency Statement|Sitemap|About US| Contact US: help@patsnap.com