A method and device for classification of iris flowers based on evolvable spiking neural network
A technology of pulse neural network and classification method, which is applied in the field of iris flower classification, can solve the problem of no effective algorithm, etc., and achieve the effect of avoiding over-training, speeding up the evolution of the network, and avoiding unnecessary updates
- Summary
- Abstract
- Description
- Claims
- Application Information
AI Technical Summary
Problems solved by technology
Method used
Image
Examples
Embodiment 1
[0041] This embodiment discloses a method for constructing an evolvable spiking neural network, including:
[0042] Step 1: Initialize the evolvable spiking neural network;
[0043] Specifically, the neural network includes an input layer, a supervisory neuron and an output layer; the maximum number of output neurons of the network is set, and the number of output types is determined according to the input training samples; and other parameters in the evolvable spiking neural network are initialized at the same time.
[0044] Such as figure 1 As shown, the structure is divided into input layer, supervisory neuron and output layer, and the input of spiking neural network is x={x 1 ,...,x i ,...,x m}, the output is Y={Y 1 ,...,Y j ,...,Y k}, the supervisory neuron is located between the input layer and the output layer, and is used to update the network weights according to the existing characteristics of the network. The supervisory neuron provides a correction parameter...
Embodiment 2
[0084] The purpose of this embodiment is to provide a computing device.
[0085] An evolvable spiking neural network construction device, comprising a memory, a processor, and a computer program stored on the memory and operable on the processor, and the processor implements the following steps when executing the program, including:
[0086] Step 1: Initialize the evolvable spiking neural network;
[0087] Step 2: Use the training samples to train the evolvable spiking neural network, and calculate the corresponding moment when the sample generates a spike in the post-synaptic potential time region;
[0088] Step 3: Evolve the neural network based on the moment selection network evolution strategy, the network evolution strategy includes adding output neuron strategy, canceling input pulse sequence training strategy and weight parameter updating strategy.
Embodiment 3
[0090] The purpose of this embodiment is to provide a computer-readable storage medium.
[0091] A computer-readable storage medium, on which a computer program is stored, and when the program is executed by a processor, the following steps are performed:
[0092] Step 1: Initialize the evolvable spiking neural network;
[0093] Step 2: Use the training samples to train the evolvable spiking neural network, and calculate the corresponding moment when the sample generates a spike in the post-synaptic potential time region;
[0094] Step 3: Evolve the neural network based on the moment selection network evolution strategy, the network evolution strategy includes adding output neuron strategy, canceling input pulse sequence training strategy and weight parameter updating strategy.
[0095] The steps involved in the devices of the above embodiments 2 and 3 correspond to those of the method embodiment 1, and for specific implementation methods, please refer to the relevant descrip...
PUM
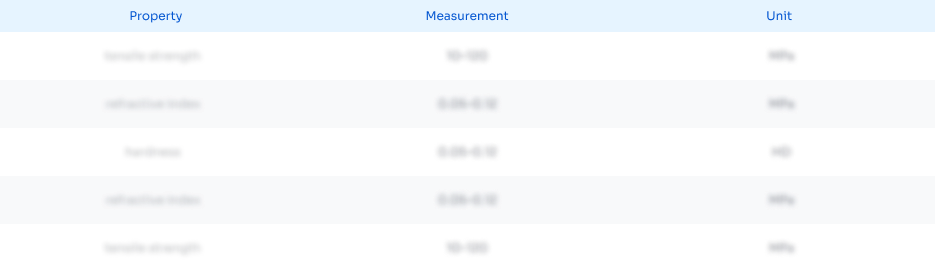
Abstract
Description
Claims
Application Information
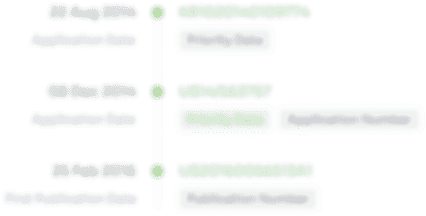
- R&D Engineer
- R&D Manager
- IP Professional
- Industry Leading Data Capabilities
- Powerful AI technology
- Patent DNA Extraction
Browse by: Latest US Patents, China's latest patents, Technical Efficacy Thesaurus, Application Domain, Technology Topic, Popular Technical Reports.
© 2024 PatSnap. All rights reserved.Legal|Privacy policy|Modern Slavery Act Transparency Statement|Sitemap|About US| Contact US: help@patsnap.com