Improving Accuracy and Reducing Complexity of Sparse Image Reconstruction Using Structural Prior Constraint
A sparse image, reconstruction accuracy technology, applied in the field of signal processing, can solve the problems of limited reconstruction accuracy, high storage complexity and time complexity
- Summary
- Abstract
- Description
- Claims
- Application Information
AI Technical Summary
Problems solved by technology
Method used
Image
Examples
Embodiment Construction
[0098] The specific implementation manners of the present invention will be further described in detail below in conjunction with the accompanying drawings and embodiments. The following examples serve to illustrate the present invention, but do not limit the scope of the present invention.
[0099] Such as figure 1 Shown, the object of the present invention is realized by following technical scheme: input observation matrix, the matrix that multi-observation vector forms, wavelet transform layer number, every layer wavelet coefficient number; Initialize system model parameter, image wavelet coefficient, maximum number of iterations , the accuracy performance index; the wavelet coefficients of the image are reconstructed by Gibbs sampling based on the Markov chain Monte Carlo method, and then the wavelet inverse transform is performed on the reconstructed wavelet coefficient matrix to obtain the reconstructed image. The present invention can apply the required precision perfo...
PUM
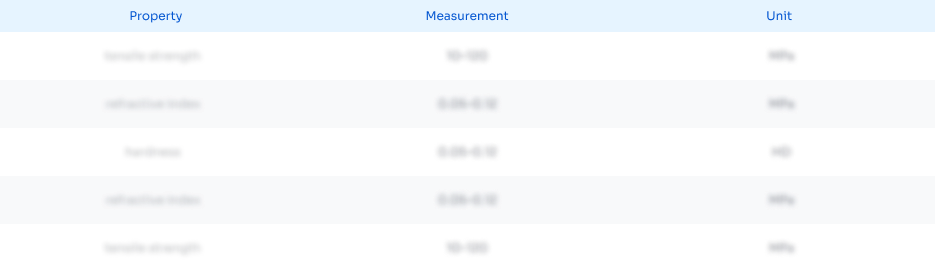
Abstract
Description
Claims
Application Information
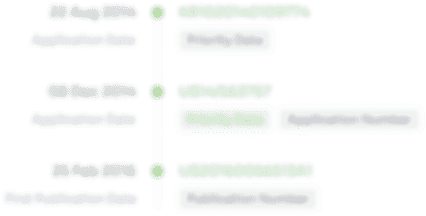
- R&D Engineer
- R&D Manager
- IP Professional
- Industry Leading Data Capabilities
- Powerful AI technology
- Patent DNA Extraction
Browse by: Latest US Patents, China's latest patents, Technical Efficacy Thesaurus, Application Domain, Technology Topic.
© 2024 PatSnap. All rights reserved.Legal|Privacy policy|Modern Slavery Act Transparency Statement|Sitemap