Bone age mark identification assessment method and system based on deep learning and image omics
A technology of deep learning and radiomics, applied in medical informatics, character and pattern recognition, instruments for radiological diagnosis, etc., can solve the problem of small amount of training data
- Summary
- Abstract
- Description
- Claims
- Application Information
AI Technical Summary
Problems solved by technology
Method used
Image
Examples
Embodiment 1
[0067] Such as figure 1 As shown, the bone age marking method based on deep learning and radiomics of this embodiment includes:
[0068] 101. Input the wrist bone image data to be marked into the RCNN-based BoneNet model, the BoneNet model includes a ResNet network structure, and the ResNet network structure is also used for migration learning of the BoneNet model;
[0069] In practice, in view of right-handed people (right-handed type), the development of the bones of the right hand will be more mature than that of the left hand, on the contrary, for left-handed people (left-handed type), the bone development of the left hand will be more mature than that of the opposite side. Therefore, here the bone image data of the wrist, the right-handed type uses the X-ray of the left hand, and vice versa. In addition, the bone image data of the wrist conforms to the DICOM (Digital Imaging and Communications in Medicine, medical digital imaging and communication) image data format;
...
Embodiment 2
[0083] like figure 2 As shown, the bone age recognition method based on deep learning and radiomics in this embodiment includes:
[0084] 201. Obtain the wrist bone image data to be identified and the corresponding inspection report, the inspection report including the type and quantity of ossification centers;
[0085] Further, clinical information corresponding to the wrist bone image data to be identified is also obtained, and the clinical information includes age and gender;
[0086] 202. Use the BoneNet model of the bone age marking method based on deep learning and radiomics as described in Example 1 to mark the bone age feature region of the wrist bone image data to be identified;
[0087] Further, the BoneNet model also includes a bone age assessment classifier, the bone age assessment classifier uses a stacking ensemble method, the stacking ensemble method includes random forest, KNN, boosting algorithm, the random forest, the KNN, the boosting Algorithms are used ...
Embodiment 3
[0096] like image 3 As shown, the bone age assessment method based on deep learning and radiomics of this embodiment includes:
[0097] 301. Obtain the bone image data of the wrist to be evaluated and corresponding clinical information, where the clinical information includes age and gender;
[0098] 302. Use the BoneNet model of the bone age recognition method based on deep learning and radiomics as described in Example 2 to locate and classify the wrist to be evaluated according to the bone image data of the wrist to be evaluated and corresponding clinical information The bone age characteristic area and the corresponding ossification center and bone quantity of the internal bone image data;
[0099] 303. Output the bone age data corresponding to the bone image data of the wrist to be evaluated, the bone age data includes the bone age characteristic area, the corresponding ossification center, the number of bones, and the bone age evaluation value, and the bone age evaluat...
PUM
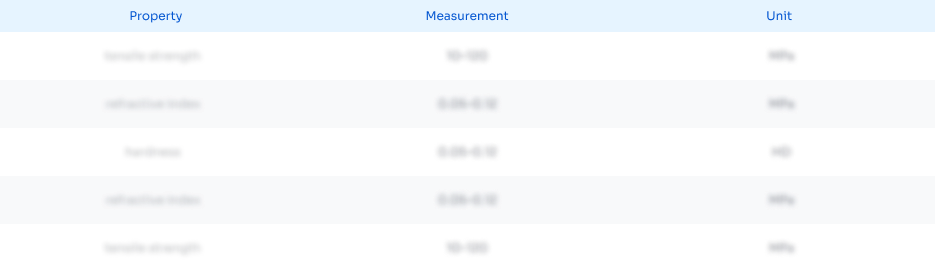
Abstract
Description
Claims
Application Information
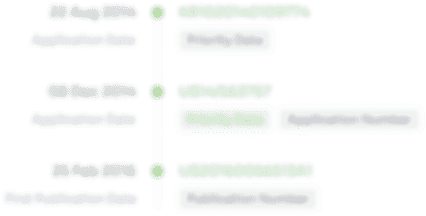
- R&D Engineer
- R&D Manager
- IP Professional
- Industry Leading Data Capabilities
- Powerful AI technology
- Patent DNA Extraction
Browse by: Latest US Patents, China's latest patents, Technical Efficacy Thesaurus, Application Domain, Technology Topic, Popular Technical Reports.
© 2024 PatSnap. All rights reserved.Legal|Privacy policy|Modern Slavery Act Transparency Statement|Sitemap|About US| Contact US: help@patsnap.com