Method of deep neural network based on discriminable region for dish image classification
A deep neural network and image technology, applied in the field of convolutional neural networks, can solve the problems of high similarity between classes, great difficulty in classifying dishes, and large differences within classes, so as to improve training efficiency, verification effectiveness and Real-time performance, the effect of improving network convergence speed
- Summary
- Abstract
- Description
- Claims
- Application Information
AI Technical Summary
Problems solved by technology
Method used
Image
Examples
Embodiment Construction
[0025] The present invention can be mainly divided into two parts: the deep neural network based on the discriminable area is used for the learning and testing of dish image classification, and the whole work can be divided into the following 5 steps:
[0026]Step 1. Build the database: First, we built a database of 90 common and popular dishes including braised pork, pork ribs and radish soup, twice-cooked pork, fried potato shreds, tomato scrambled eggs, minced meat eggplant, fried broccoli, etc. Image database, 1500 images per category, samples of these images are from recipe websites. We randomly select 1200 of them as training samples, and the remaining 300 as testing samples. All image sizes are normalized. Due to the large number of network parameters and the small number of samples, in order to avoid overfitting, the images randomly cropped from the images during training are used for network training to increase the number of samples.
[0027] Step 2. Classify the d...
PUM
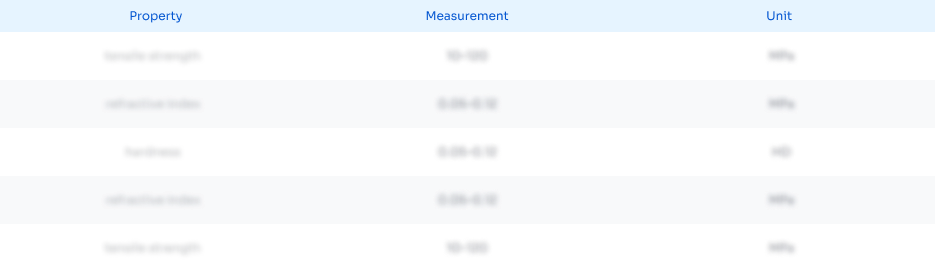
Abstract
Description
Claims
Application Information
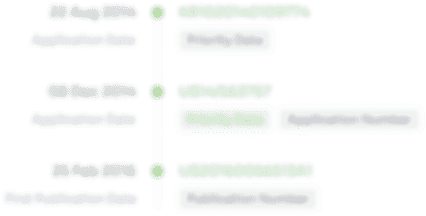
- R&D Engineer
- R&D Manager
- IP Professional
- Industry Leading Data Capabilities
- Powerful AI technology
- Patent DNA Extraction
Browse by: Latest US Patents, China's latest patents, Technical Efficacy Thesaurus, Application Domain, Technology Topic, Popular Technical Reports.
© 2024 PatSnap. All rights reserved.Legal|Privacy policy|Modern Slavery Act Transparency Statement|Sitemap|About US| Contact US: help@patsnap.com