Feature loss-based medical image super-resolution reconstruction method
A technology for super-resolution reconstruction and medical image, applied in the field of medical image super-resolution reconstruction based on feature loss, which can solve problems such as checkerboard and lattice artifacts, and achieve a wide range of applications.
- Summary
- Abstract
- Description
- Claims
- Application Information
AI Technical Summary
Problems solved by technology
Method used
Image
Examples
Embodiment 1
[0030] A Feature Loss-Based Method for Medical Image Super-Resolution Reconstruction, Including Image Transformation Network f w , the image conversion network f w It is a feedforward fully connected neural network. The feedforward neural network divides each neuron in the network into different groups according to the order of receiving information. Each group is regarded as a network layer, and the neurons in each layer receive The number output of the previous layer of neurons is used as its own input, and then its own output is input to the next layer, and the information in the entire network is propagated in one direction; the image conversion network f w Process a low-resolution medical image of size H / 4×W / 4, and convert the low-resolution medical image of size H / 4×W / 4 into a high-resolution image of size H×W, where H and W are natural numbers . The image conversion network f w Including two upscaling convolutional layers, after two upscaling convolutional layers, th...
Embodiment 2
[0032] A Feature Loss-Based Method for Medical Image Super-Resolution Reconstruction, Including Image Transformation Network f w , the image conversion network f w It is a feedforward fully connected neural network. The feedforward neural network divides each neuron in the network into different groups according to the order of receiving information. Each group is regarded as a network layer, and the neurons in each layer receive The number output of the previous layer of neurons is used as its own input, and then its own output is input to the next layer, and the information in the entire network is propagated in one direction; the image conversion network f w Process a low-resolution medical image of size H / 4×W / 4, and convert the low-resolution medical image of size H / 4×W / 4 into a high-resolution image of size H×W, where H and W are natural numbers . The image conversion network f w Including two upscaling convolutional layers, after two upscaling convolutional layers, th...
PUM
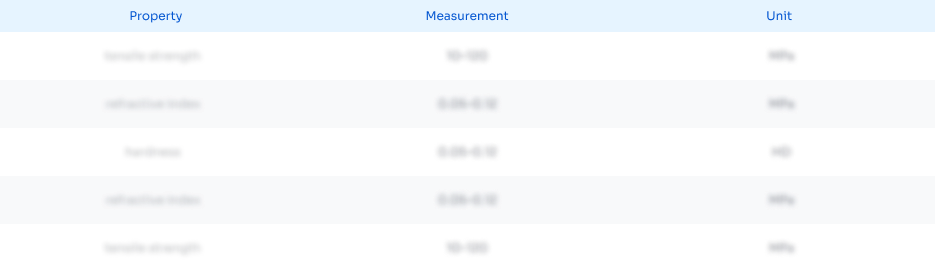
Abstract
Description
Claims
Application Information
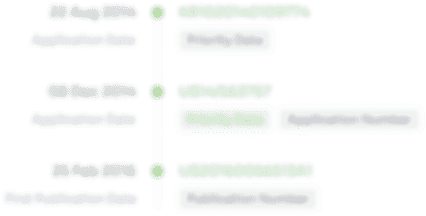
- R&D Engineer
- R&D Manager
- IP Professional
- Industry Leading Data Capabilities
- Powerful AI technology
- Patent DNA Extraction
Browse by: Latest US Patents, China's latest patents, Technical Efficacy Thesaurus, Application Domain, Technology Topic, Popular Technical Reports.
© 2024 PatSnap. All rights reserved.Legal|Privacy policy|Modern Slavery Act Transparency Statement|Sitemap|About US| Contact US: help@patsnap.com