Partitioning algorithm for choroidal neovascularization in OCT image
A new blood vessel, segmentation algorithm technology, applied in image analysis, image enhancement, image data processing and other directions, can solve the problems of affecting segmentation accuracy, not taking into account, limiting model segmentation performance, etc., to enhance correlation and improve segmentation accuracy. Effect
- Summary
- Abstract
- Description
- Claims
- Application Information
AI Technical Summary
Problems solved by technology
Method used
Image
Examples
Embodiment 1
[0041] A choroidal neovascularization segmentation algorithm in OCT images, such as figure 1 As shown, this method mainly includes two stages: training stage and testing stage, the specific steps are as follows:
[0042] (1) The training phase mainly includes two parts: "structural prior learning" and "multi-scale structural prior convolutional neural network training". The specific steps are as follows:
[0043] S01: Design a structure prior learning method for the training image, construct a structure prior matrix, and the structure prior matrix is used to distinguish the choroidal neovascularization area and the background area;
[0044] Described structure prior learning method comprises the following steps:
[0045] a: Perform superpixel segmentation on the training image to obtain several superpixel regions, and use the SLIC algorithm to segment;
[0046] b: extract features, the features include the average gray value of each superpixel, texture features based on co...
PUM
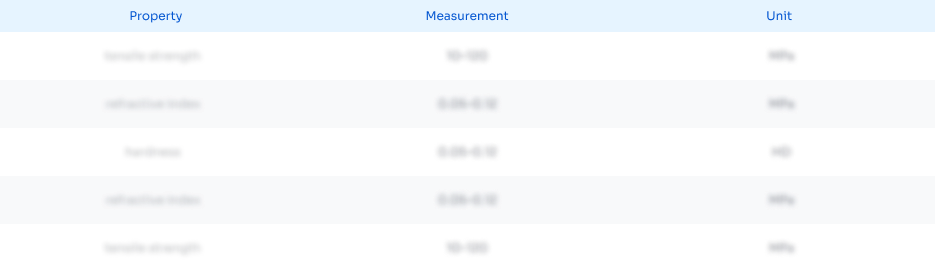
Abstract
Description
Claims
Application Information
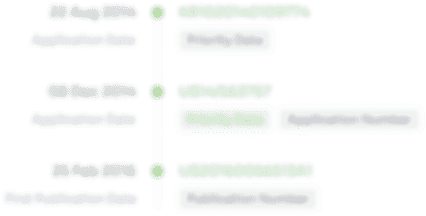
- R&D Engineer
- R&D Manager
- IP Professional
- Industry Leading Data Capabilities
- Powerful AI technology
- Patent DNA Extraction
Browse by: Latest US Patents, China's latest patents, Technical Efficacy Thesaurus, Application Domain, Technology Topic, Popular Technical Reports.
© 2024 PatSnap. All rights reserved.Legal|Privacy policy|Modern Slavery Act Transparency Statement|Sitemap|About US| Contact US: help@patsnap.com