Automatic editing-after-translating system and method for multisource neural network based on splicing-remixing mode
A post-translation editing and neural network technology, which is applied in the fields of natural language processing and machine translation, can solve problems such as missing translations, improve the overall quality, improve translation fidelity, and improve the overall translation quality
- Summary
- Abstract
- Description
- Claims
- Application Information
AI Technical Summary
Problems solved by technology
Method used
Image
Examples
Embodiment 1
[0059] This embodiment combines the attached figure 1 , describes the detailed composition, training and decoding process of a multi-source neural network post-translation editing system and method based on splicing and remixing in the present invention.
[0060] From figure 1 It can be seen that the training module is connected to the decoding module.
[0061] The training process of the training module includes the following steps:
[0062] Step A: collecting various corpus required for the training process of the system;
[0063] Wherein, each corpus mainly includes the training original text corpus and the reference translation corpus; wherein, the training original text corpus and the reference translation corpus are parallel corpora; assuming N=600000, that is, the training original text has 60,000 sentences;
[0064] Training original corpus, recorded as: {source 1 , source 2 ,..., source 600000},
[0065] Training translation corpus, denoted as {ref 1 , ref 2 ...
Embodiment 2
[0094] In this embodiment, a specific sentence is taken as an example to illustrate the effect of the system and method.
[0095] In a specific example, the quality of translation is intuitively reflected in fidelity and fluency, and the improvement of fidelity is subdivided into the improvement of word selection accuracy.
[0096] Suppose the translation of the original text is "However, the challenges in the past were not limited to funding public housing, private housing was also full of major tests." sentence.
[0097] The preliminary machine translation system uses the Moses statistical machine translation system, and the translation result is "however, the pastchallenge, not in the funding of public housing, private housing is full of challenge." In this sentence, the keyword "funding" in the original translation is replaced by Translated into "funding", which means "providing funds for...", lacks the meaning of help level, and is not accurate enough. At the same time, t...
Embodiment 3
[0101] This example illustrates in a statistical sense that this system and method are compared with the single-source neural network automatic post-translation editing system that does not add the original translation and directly uses the preliminary translation results as the source language training, and the multi-source method that only splices but does not mix. Advantages of neural network automatic post-translation editing systems in terms of overall translation quality.
[0102] Assume that there are 600,000 sentences in the training original text and reference translation data sets used for the training module, and 1597 sentences in the original translation data set used in the test module. The preliminary machine translation system uses the Moses statistical machine translation system, and the scoring uses the multi-bleu script. The BLEU value Represents the overall translation quality, and the one-to-four scores are quantitative indicators of fidelity and fluency, re...
PUM
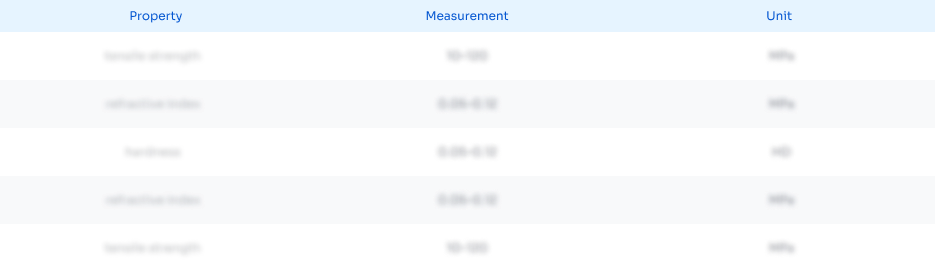
Abstract
Description
Claims
Application Information
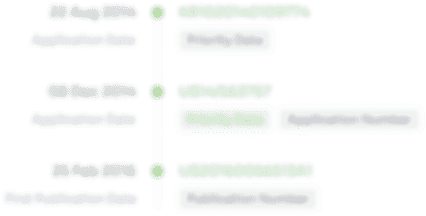
- R&D Engineer
- R&D Manager
- IP Professional
- Industry Leading Data Capabilities
- Powerful AI technology
- Patent DNA Extraction
Browse by: Latest US Patents, China's latest patents, Technical Efficacy Thesaurus, Application Domain, Technology Topic, Popular Technical Reports.
© 2024 PatSnap. All rights reserved.Legal|Privacy policy|Modern Slavery Act Transparency Statement|Sitemap|About US| Contact US: help@patsnap.com