Depth feature-based high-spatial resolution remote sensing image migration learning method
A high-spatial-resolution, deep-featured technology, applied in instruments, scene recognition, computing, etc., can solve problems such as inability to apply remote sensing images, achieve the effect of improving image transfer learning and avoiding the difference of image spectral values
- Summary
- Abstract
- Description
- Claims
- Application Information
AI Technical Summary
Problems solved by technology
Method used
Image
Examples
Embodiment Construction
[0025] The implementation of a high-spatial-resolution remote sensing image transfer learning method based on depth features by using the present invention is shown in the accompanying drawings, which will now be described in conjunction with the accompanying drawings.
[0026]The processing unit 100 performs principal component transformation on current common multi-band high-spatial-resolution remote sensing images (such as four-band IKONOS satellite multispectral images, eight-band WorldView-4 satellite multispectral images), using the first three components ( That is, the first three components with the most information) generate a three-band image.
[0027] The processing unit 101 reads image blocks of odd-numbered window sizes (such as 5×5, 7×7, 9×9, 11×11) for each pixel of the image obtained in the processing unit 100 as the geometric center, the image A block acts as a representation that the pixel has spatial context information.
[0028] The processing unit 102 inp...
PUM
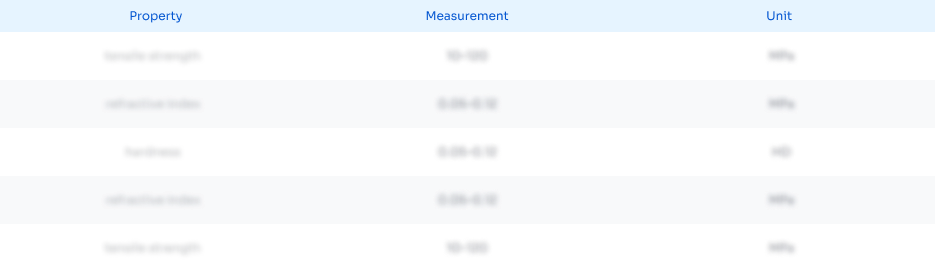
Abstract
Description
Claims
Application Information
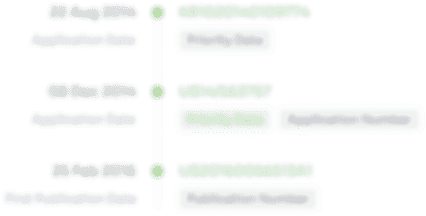
- R&D Engineer
- R&D Manager
- IP Professional
- Industry Leading Data Capabilities
- Powerful AI technology
- Patent DNA Extraction
Browse by: Latest US Patents, China's latest patents, Technical Efficacy Thesaurus, Application Domain, Technology Topic, Popular Technical Reports.
© 2024 PatSnap. All rights reserved.Legal|Privacy policy|Modern Slavery Act Transparency Statement|Sitemap|About US| Contact US: help@patsnap.com