Power system dynamic state estimation method based on singular value decomposition CDKF
A technology of dynamic state estimation and singular value decomposition, applied in computing, instrumentation, data processing applications, etc., can solve the problems of inaccurate process noise covariance, loss of positive definite covariance matrix, large amount of calculation, etc., to improve filtering accuracy, Enhanced numerical stability and simple parameter adjustment
- Summary
- Abstract
- Description
- Claims
- Application Information
AI Technical Summary
Problems solved by technology
Method used
Image
Examples
Embodiment
[0082] Calculation example of the present invention test
[0083] The method of the invention is tested on IEEE14 node and IEEE30 node systems. Firstly, the power flow calculation program is used to obtain the power flow calculation results as the real value, and the random error of the power flow true value superimposed to obey the normal distribution is sent to the state estimator as the measured value. Wherein, the standard deviation of the power type measurement is set to 2% of its true value, and the standard deviation of the voltage amplitude measurement is set to 1% of its true value. The load fluctuation and power output fluctuation curves come from the daily record data of a power dispatching center, and the data curves under the sampling methods of 24 points (60min sampling), 96 points (15min sampling) and 144 points (10min sampling) were obtained respectively.
[0084] In order to verify the performance of the method of the present invention, the performance of the...
PUM
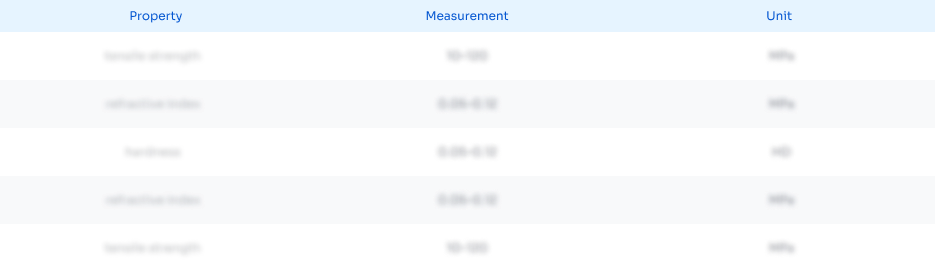
Abstract
Description
Claims
Application Information
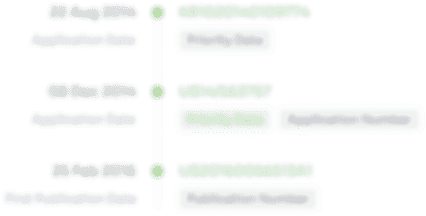
- R&D Engineer
- R&D Manager
- IP Professional
- Industry Leading Data Capabilities
- Powerful AI technology
- Patent DNA Extraction
Browse by: Latest US Patents, China's latest patents, Technical Efficacy Thesaurus, Application Domain, Technology Topic.
© 2024 PatSnap. All rights reserved.Legal|Privacy policy|Modern Slavery Act Transparency Statement|Sitemap