Stacked SAE (Sparse Autoencoder) deep neural network-based bearing fault diagnosis method
A deep neural network, fault diagnosis technology, applied in neural learning methods, biological neural network models, mechanical bearing testing, etc., can solve problems such as not too much
- Summary
- Abstract
- Description
- Claims
- Application Information
AI Technical Summary
Problems solved by technology
Method used
Image
Examples
Embodiment Construction
[0082] The specific embodiments of the present invention will be described in further detail below with reference to the accompanying drawings and embodiments. The following examples are intended to illustrate the present invention, but not to limit the scope of the present invention.
[0083] The present invention is described in detail below in conjunction with actual experimental data:
[0084] The experimental data adopts the bearing data set of Case Western Reserve University, which contains a total of 4 fault types: inner ring fault, rolling element fault, outer ring fault and normal state, respectively define their ideal labels as 1, 2, 3, 4, The time domain waveform is shown as image 3 shown. The fault size in each fault state includes 3 sizes: 0.007 inches, 0.014 inches and 0.021 inches, and the respective labels are defined as 1, 2, and 3, so there are 3*3+1=10 operating states in total. The sampling frequency is 12KHz, 600 signal samples are sampled in each oper...
PUM
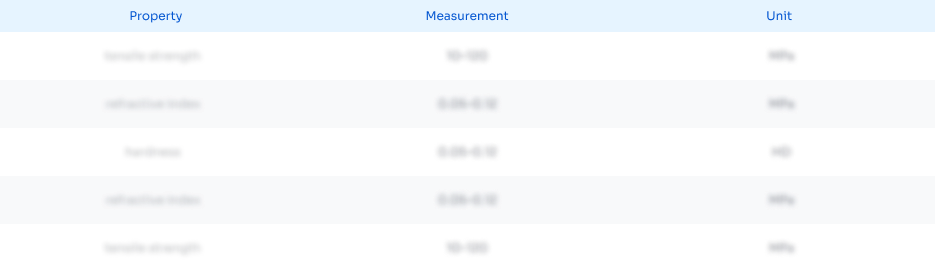
Abstract
Description
Claims
Application Information
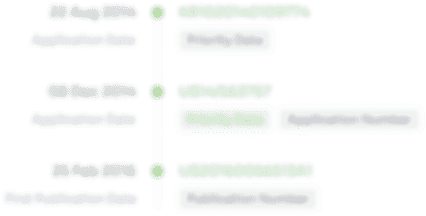
- R&D Engineer
- R&D Manager
- IP Professional
- Industry Leading Data Capabilities
- Powerful AI technology
- Patent DNA Extraction
Browse by: Latest US Patents, China's latest patents, Technical Efficacy Thesaurus, Application Domain, Technology Topic, Popular Technical Reports.
© 2024 PatSnap. All rights reserved.Legal|Privacy policy|Modern Slavery Act Transparency Statement|Sitemap|About US| Contact US: help@patsnap.com