A Single-bit Spatial Spectrum Estimation Method Based on Support Vector Machine
A space spectrum estimation and support vector machine technology, applied in the field of support vector machines, can solve problems such as large amount of calculation and poor accuracy, and achieve the effects of low requirements, reduced cost and complexity, and good angle estimation accuracy
- Summary
- Abstract
- Description
- Claims
- Application Information
AI Technical Summary
Problems solved by technology
Method used
Image
Examples
specific Embodiment approach 1
[0055] Specific implementation mode one: see figure 1 Describe this embodiment mode, a kind of single-bit spatial spectrum estimation method based on logistic regression described in this embodiment mode, this method comprises the following steps:
[0056] Step 1: Construct a sample training model according to the single-bit received data;
[0057] Step 2: For the input and output of the construction sample training model, use the support vector machine algorithm to calculate the classification coefficient vector t, where t=[t 1 ,t 2 ,...,t i ,...,t 2m ] T ;
[0058] Step 3: According to the classification coefficient vector t and the following formula 1:
[0059] S i = t i +j×t i+m(Formula 1);
[0060] Obtain the spatial spectrum S=[S 1 ,S 2 ,...,S m ] T , so as to complete the estimation of the spatial spectrum S;
[0061] Among them, i and m are both integers, t i is the ith component of the classification coefficient vector t, t i+m is the i+mth component ...
specific Embodiment approach 2
[0071] Specific implementation mode two: see figure 1 Describe this embodiment. The difference between this embodiment and the method for estimating a single-bit spatial spectrum based on a support vector machine described in the first embodiment is that in the first step, the sample training model is constructed according to the single-bit received data. The specific process is:
[0072] Step one, train the model on the original sample:
[0073]
[0074] Perform sparse representation and obtain the original sample training model after sparse representation:
[0075] x=FS (Formula 3),
[0076] Steps 1 and 2, perform single-bit quantization on the original sample training model after sparse representation, and obtain the model after single-bit quantization:
[0077]
[0078] In step 13, the single-bit quantized model is expressed in the real number domain as,
[0079] q=sign(Φt+e′) (Formula 5),
[0080] The single-bit quantized model is a sample training model constr...
specific Embodiment approach 3
[0101] Specific embodiment three: the difference between this embodiment and the single-bit spatial spectrum estimation method based on support vector machine described in specific embodiment one or two is that the output of the constructed sample training model is the observation vector q, and the constructed sample The input to the training model is the row of the flow pattern matrix Φ.
PUM
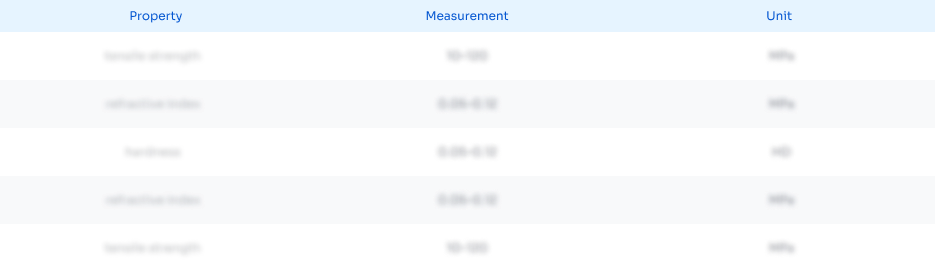
Abstract
Description
Claims
Application Information
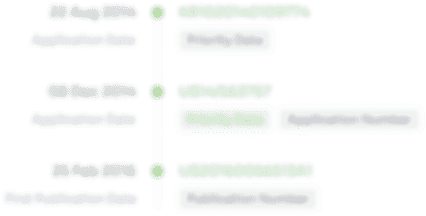
- R&D Engineer
- R&D Manager
- IP Professional
- Industry Leading Data Capabilities
- Powerful AI technology
- Patent DNA Extraction
Browse by: Latest US Patents, China's latest patents, Technical Efficacy Thesaurus, Application Domain, Technology Topic, Popular Technical Reports.
© 2024 PatSnap. All rights reserved.Legal|Privacy policy|Modern Slavery Act Transparency Statement|Sitemap|About US| Contact US: help@patsnap.com