Large data volume prediction three-layer combined dynamic selection optimal model method
A forecasting model and large data volume technology, applied in forecasting, data processing applications, calculations, etc., can solve problems such as spending a lot of time, wrong model selection, poor forecasting effect, etc., to achieve accuracy, high scalability, and guaranteed data The effect of length
- Summary
- Abstract
- Description
- Claims
- Application Information
AI Technical Summary
Problems solved by technology
Method used
Image
Examples
Embodiment
[0044] Such as figure 1 , the three-layer joint dynamic selection optimal model method for large data volume prediction consists of three layers: a prediction model algorithm library, a weight algorithm library, and an optimal weight algorithm selection algorithm.
[0045] The prediction model algorithm library includes various classic algorithms, improved classic algorithms and some patented algorithms. These algorithms are abstracted into common interfaces and placed at the bottom of the joint algorithm to provide prediction functions and support higher-level functions.
[0046] On top of the prediction algorithm model library is the weight algorithm. The weight algorithm packs the prediction algorithm library to shield the diversity of the bottom-level algorithms. Users do not need to consider the parameters, cycle, convergence, error, etc. of the bottom-level algorithms. , the weighting algorithm is based on the prediction results of the underlying algorithm, according to ...
PUM
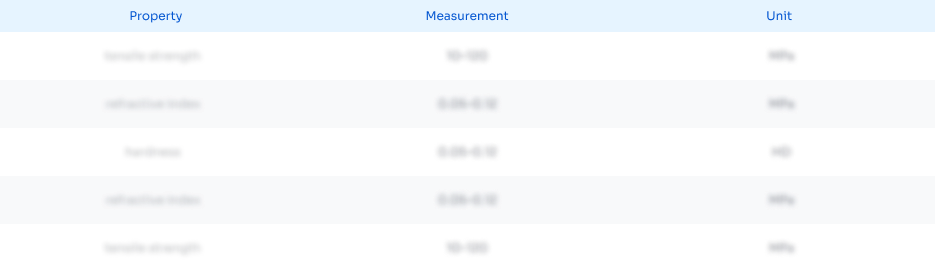
Abstract
Description
Claims
Application Information
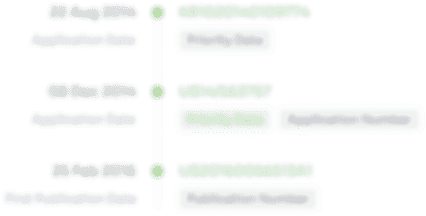
- R&D Engineer
- R&D Manager
- IP Professional
- Industry Leading Data Capabilities
- Powerful AI technology
- Patent DNA Extraction
Browse by: Latest US Patents, China's latest patents, Technical Efficacy Thesaurus, Application Domain, Technology Topic, Popular Technical Reports.
© 2024 PatSnap. All rights reserved.Legal|Privacy policy|Modern Slavery Act Transparency Statement|Sitemap|About US| Contact US: help@patsnap.com