Deep extreme learning machine-based hazard source identification method
A technology of extreme learning machine and recognition method, which is applied in the direction of neural learning method, character and pattern recognition, computer parts, etc., can solve the problems of complexity, expansive flexibility of experience database, and inability to make full use of it, and achieve the goal of slowing down the growth rate Effect
- Summary
- Abstract
- Description
- Claims
- Application Information
AI Technical Summary
Problems solved by technology
Method used
Image
Examples
Embodiment Construction
[0035] The technical solutions of the present invention will be described in detail below in conjunction with the accompanying drawings.
[0036] The present invention provides a hazard identification method based on a deep extreme learning machine. First, the heterogeneous deep neural network used is described, as follows figure 1 As shown, the neural network is composed of two parts. The first part is a deep structure organized according to modules. This structure is composed of multiple deep neural networks. According to different input data, these deep neural networks can have different numbers of hidden nodes and The number of layers; the second part is a single hidden layer neural network, which is used to receive the learned features of the deep structure.
[0037] (1) Deep structure module
[0038] In the deep structure, each module can be divided according to the field of hazard state information or other classification rules of hazard state information, and differen...
PUM
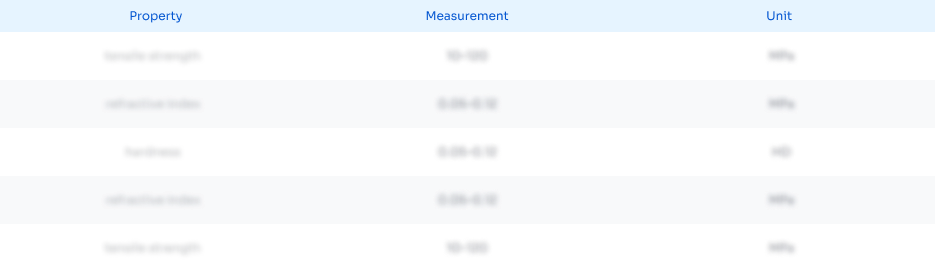
Abstract
Description
Claims
Application Information
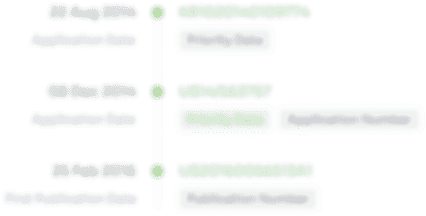
- Generate Ideas
- Intellectual Property
- Life Sciences
- Materials
- Tech Scout
- Unparalleled Data Quality
- Higher Quality Content
- 60% Fewer Hallucinations
Browse by: Latest US Patents, China's latest patents, Technical Efficacy Thesaurus, Application Domain, Technology Topic, Popular Technical Reports.
© 2025 PatSnap. All rights reserved.Legal|Privacy policy|Modern Slavery Act Transparency Statement|Sitemap|About US| Contact US: help@patsnap.com