Short-term traffic flow prediction method based on nerve network combination model
A traffic flow and neural network technology, which is applied in the field of short-term traffic flow forecasting, can solve the problems of large impact on forecasting accuracy, unreasonable running time, and low short-term traffic flow forecasting accuracy.
- Summary
- Abstract
- Description
- Claims
- Application Information
AI Technical Summary
Problems solved by technology
Method used
Image
Examples
Embodiment Construction
[0039] The present invention is aimed at short-term traffic flow prediction at crossroads, and considers the diversity of traffic flow distribution forms, model training adequacy and prediction accuracy in specific implementation, and reduces random generation or artificial designation of these parameter values. The present invention will be described in more detail below.
[0040] In the specific implementation of the present invention, a backpropagation neural network combination prediction model is first constructed, which is composed of a backpropagation neural network prediction model, a fuzzy C-means clustering model and a structural parameter selection model.
[0041] The backpropagation neural network is a multi-layer feedforward neural network, and its main feature is that the signal propagates forward and the error propagates backward. In the forward propagation, the input signal is processed layer by layer from the input layer through the hidden layer to the output ...
PUM
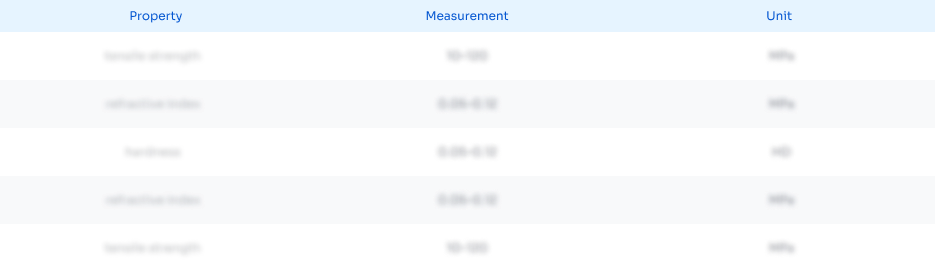
Abstract
Description
Claims
Application Information
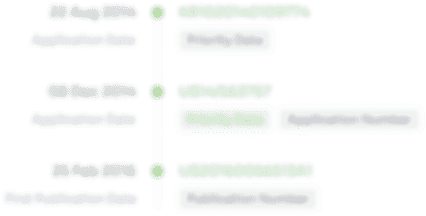
- R&D Engineer
- R&D Manager
- IP Professional
- Industry Leading Data Capabilities
- Powerful AI technology
- Patent DNA Extraction
Browse by: Latest US Patents, China's latest patents, Technical Efficacy Thesaurus, Application Domain, Technology Topic, Popular Technical Reports.
© 2024 PatSnap. All rights reserved.Legal|Privacy policy|Modern Slavery Act Transparency Statement|Sitemap|About US| Contact US: help@patsnap.com